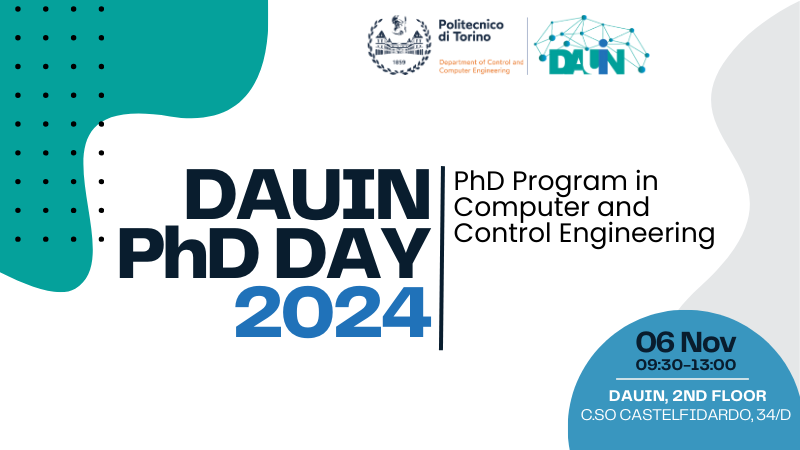
On Wednesday, November 6, 2024, on the second floor of the Department of Control and Computer Engineering (DAUIN), the Ph.D. students enrolled in the 2nd and 3rd year of the Ph.D. Program in Computer and Control Engineering (37th and 38th cycle) will have a chance to present and discuss in person their research activities in the context of the 8th edition of the DAUIN Ph.D. Day. The event will be an opportunity to illustrate the Ph.D. Programs managed by the Department and disseminate the research activities that are carried out by its staff. The students will be available to share their experience and research activities through posters and demo presentations.
Agenda
Welcome (9:30 – 9:50)
Prof. Luca Sterpone, Head of the Department of Control and Computer Engineering
Prof. Stefano Grivet Talocia, Head of the Doctoral School
Prof. Fabrizio Lamberti, Coordinator of the PhD Programme in Computer and Control Engineering
Prof. Stefano di Carlo, Coordinator of the National PhD Programme in Artificial Intelligence – Industry
PhD quality awards: The top PhD students 2024 will be recognized for their outstanding achievements and results.
- 36th cycle IIS: Francesco Daghero, Chiara Plizzari, Juan David Guerrero Balaguera
- 37th cycle IIS: Gianluca Amprimo, Antonino Angi, Gabriele Moreno Berton, Emanuele Dri, Tommaso Fulcini, Simone Monaco, Pietro Rando Marazzino, Matteo Risso
- 37th cycle AI: Jonathan Melchiorre
Testimonials (9:50 – 10:00)
Dr. Luigi Borzì, former student (35th cycle), now a Research Assistant (RTD-A) at the Department of Control and Computer Engineering
Dr. Eliana Giovannitti, former student (34th cycle), now Analytics Engineer at COMAU
Ph.D. Poster/Demo presentations and discussion (10:00 – 12:45)
During the session, professors and past Ph.D. students will be available to provide information about the Ph.D. Program, admission, as well as formative and research activities.
Awards ceremony (12:45 – 13:00)
Best poster and best demo awards ceremony.
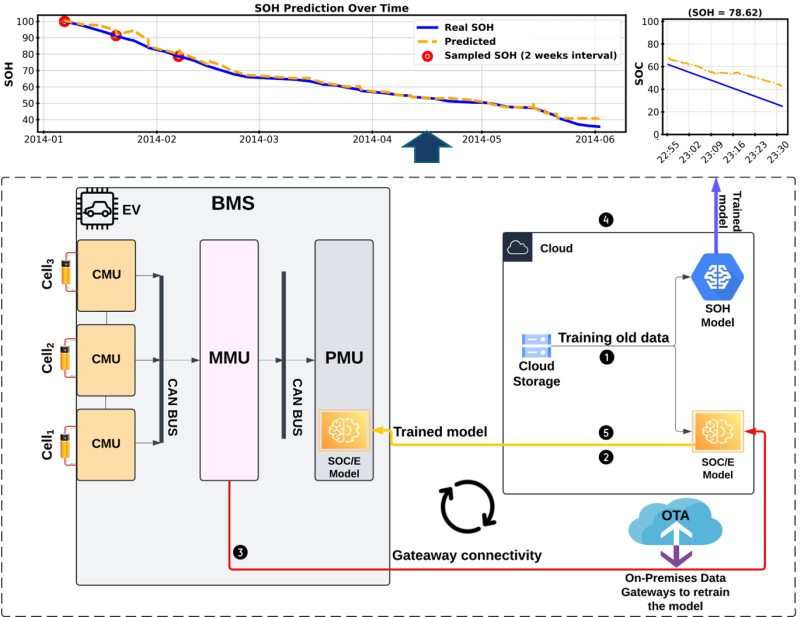
Innovative AI-Enabled Digital Twins for Smart Battery Management: Enhancing Battery Life and Performance in Smart Vehicles
This research advances battery management systems (BMS) by using AI-driven digital twin technology. It combines data-driven methods, machine learning, and cloud-edge connectivity to continuously update models of battery health (SOH) and charge (SOC). This approach optimizes battery performance in real-time, improving electric vehicle reliability and longevity, transforming battery management for software-defined vehicles.
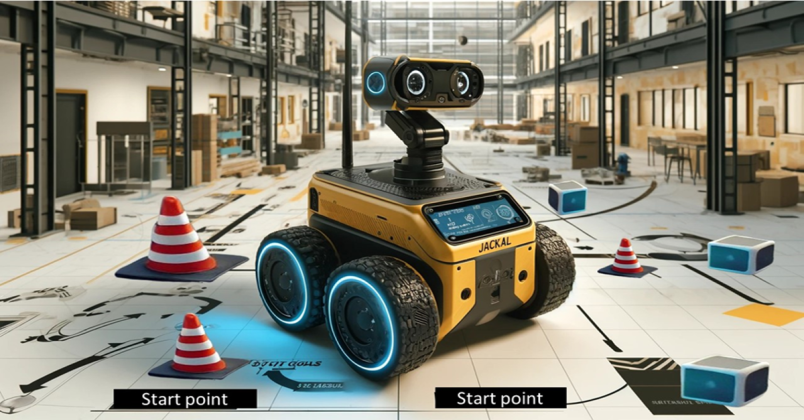
Advanced Navigation Control Systems for Autonomous Vehicles
Our research focuses on developing advanced control systems for mobile robots to navigate from a starting position to a goal position in environments with complexities. Using deep reinforcement learning, we aim to optimize the robot's path to maximize success rates, minimize collisions, and ensure timely navigation. This involves creating algorithms that enable the robot to learn and adapt to dynamic changes in real-time. Our goal is to contribute to safer and more efficient autonomous transportation systems, pushing the boundaries of what autonomous vehicles can achieve in complex environments.
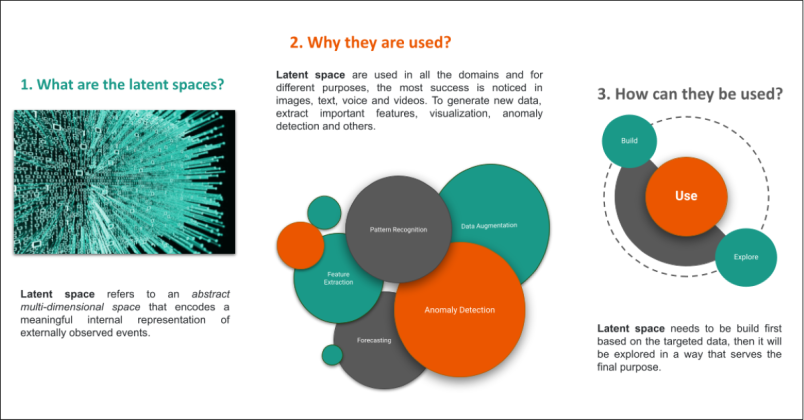
Exploring The Latent Space for Machine Learning Applications - Security Challenges
The research focuses on exploring latent spaces to improve understanding of AI models' behavior. The main directions are the Theory of building these spaces as estimation of real-world data, the Methodologies to explore their properties (such as projection, exploration, visualization, and others) and the possible real-world Applications to generalize the obtained results.
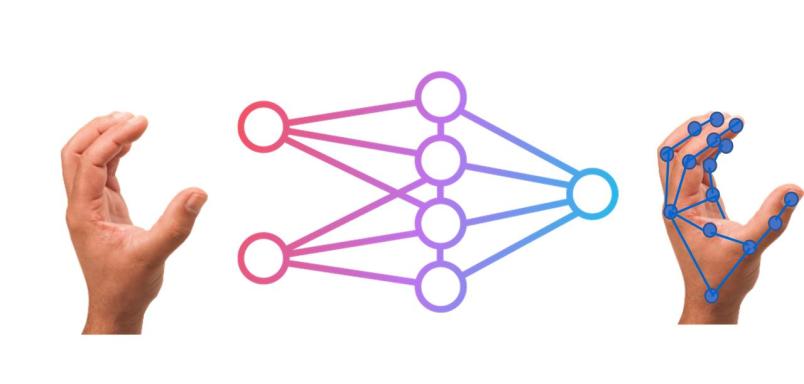
Vision-based, AI-powered approaches to motor functions characterization and rehabilitation
This PhD project explores the intersection of computer vision and artificial intelligence for the automatic and quantitative characterization of human movement using video-based body pose estimation. Additionally, these technologies are used as human-computer interaction means in exergames for gamified rehabilitation and assessment, suitable for telemedicine systems.
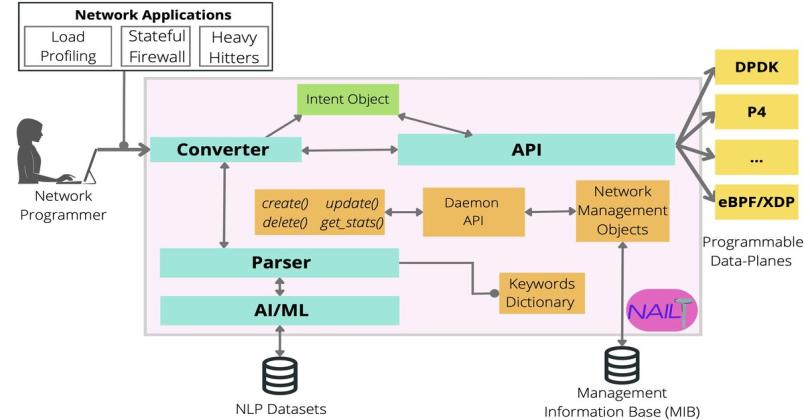
Integration of ML and NFV for the Management of Distributed Edge Infrastructures
My research focuses on integrating Natural Language Processing, Large Language Models, and Machine Learning algorithms with Software Defined Networks and Intent-based Networks. The goal is to enhance network automation and intelligence using protocols such as OpenFlow and data-plane programming languages such as P4, also allowing more efficient, responsive, and adaptable management of the network.
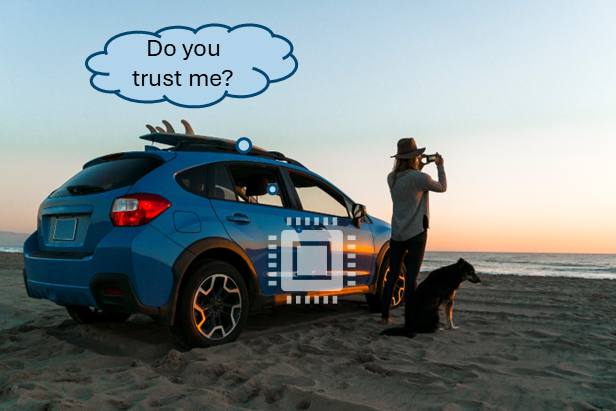
System-Level-Test Techniques for Automotive SoCs
This research investigates System-Level Test (SLT) functional techniques for Automotive SoCs during manufacturing test flow for further increasing quality of semiconductor products. It proposes methodologies for evaluating SLT techniques using a comprehensive test setup that spans various design stages (layout, RTL, code) and data sources (silicon measurements, instruction traces, high-level models). The focus extends beyond core processors to encompass peripherals like timers, communication interfaces, and machine learning accelerators, while also exploring crossbar-specific testing approaches.
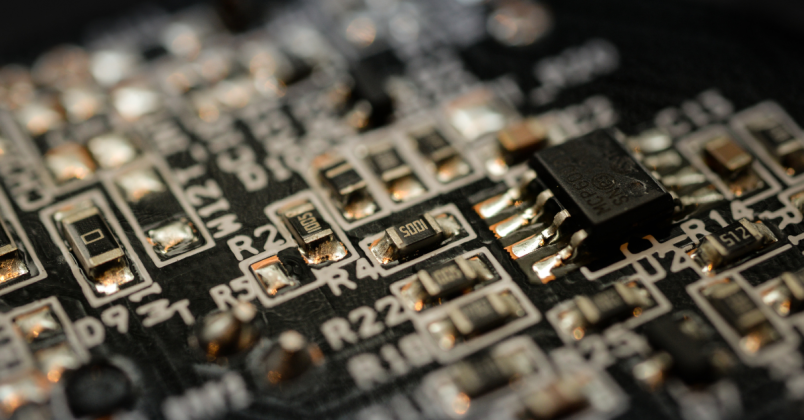
Computational Intelligence for Computer-Aided Design
Research focuses on developing machine learning (ML)-based methods for microcontroller performance screening. Traditional approaches, based on system-level tests, are time-consuming and limited by application diversity. By using indirect performance monitoring structures like ring oscillators, coupled with ML, it is possible to accurately estimate chip performance from data, ensuring efficient and reliable screening.
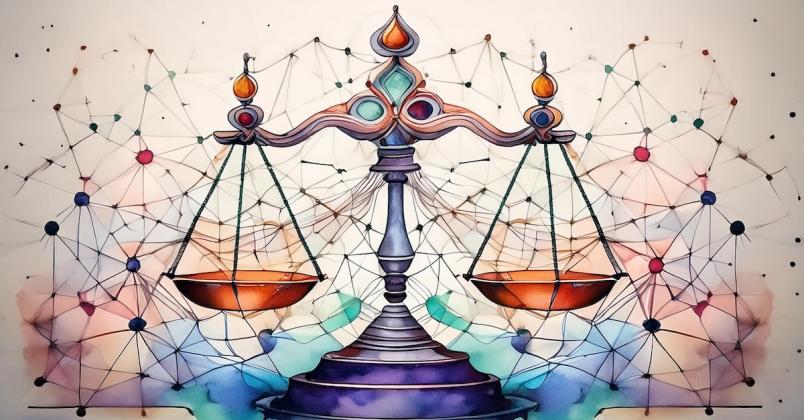
Enhancing Decision-Making with Large Language Models in the Legal Domain
Her research focuses on developing innovative solutions in the legal field to enhance content accessibility and expedite decision-making processes using large language models (LLMs). This includes the advancement of state-of-the-art methodologies for named entity recognition, document classification, Explainable AI (XAI), and reasoning applied to the legal domain.
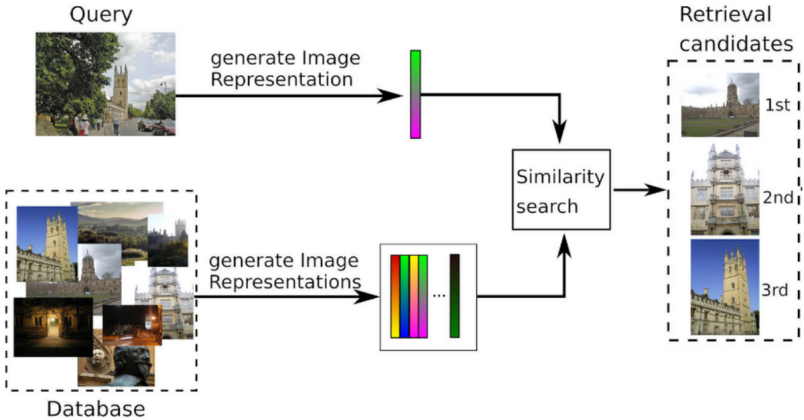
Large-scale visual place recognition
The goal of my research is to be able to confidently and quickly identify the location depicted within a given photo, by comparing it with a database of images of known locations. Such research finds applications in robotics, 3D reconstructions, astronaut photography localization and disaster management.
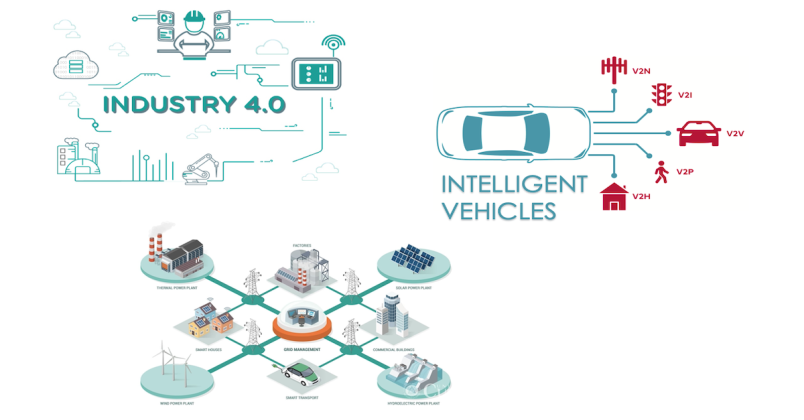
Formal Techniques for Cyber-Physical Systems
The goal of my PhD is to explore the potential of formal methods when applied to cybersecurity management and security verification of cyber-physical systems. On the one hand, I focus on solutions to automatically allocate and configure appropriate security mechanisms to protect a CPS. On the other hand, I focus on the formal verification of these systems from a security and privacy perspective.
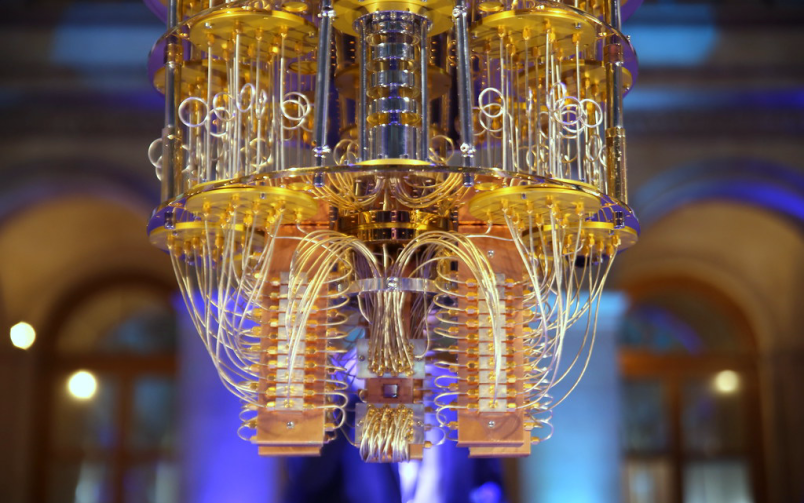
Quantum Computing for Finance
The research work explores leveraging quantum computing (QC) to solve complex financial problems. We aim to analyze and improve current quantum finance algorithms to make them practical for real-world use, partnering with Intesa Sanpaolo and IBM Consulting. Key results include enhanced credit risk analysis, practical implementation of quantum PCA, and extending quantum derivative pricing algorithms.
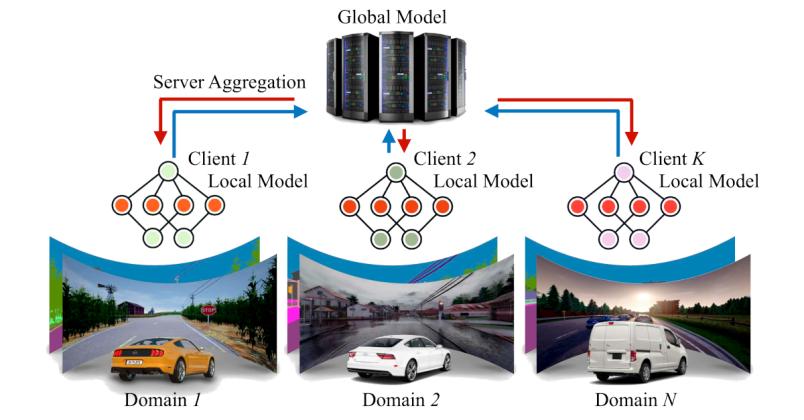
Addressing Heterogeneity in Federated Learning for Real-world Vision Applications
Federated Learning enables training models on edge devices while preserving the user’s privacy. However, non-IID data can lead to biased model training and slow convergence speed. This research addresses the FL challenges in real-world vision applications, identifying the factors that hinder the training process and the potential issues related to the specific tasks and scenarios.
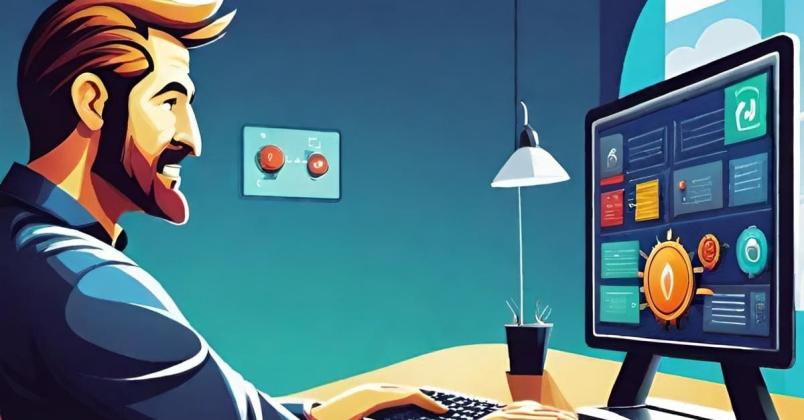
Gamification of E2E Software
Gamification is defined as the application of game elements in a non-gaming context. Software testing is a discipline often considered boring and uninspiring. This PhD program aims to create a playful environment to support testers during the testing activities, spanning from unit testing to End-to-end testing, to improve their UX by achieving higher user involvement and better performance.
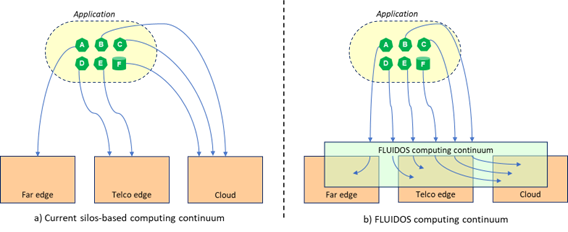
Distributed orchestration in a computing continuum
Edge and fog computing are bringing the advantages of cloud technologies at the edge of the network. In the near feature we expect to include also customer devices in this computing continuum, creating the so called “liquid computing” in which tasks can be executed almost anywhere. The current PhD is oriented to investigate the continuum and its applications with a focus on sustainable computing and energy and battery management.
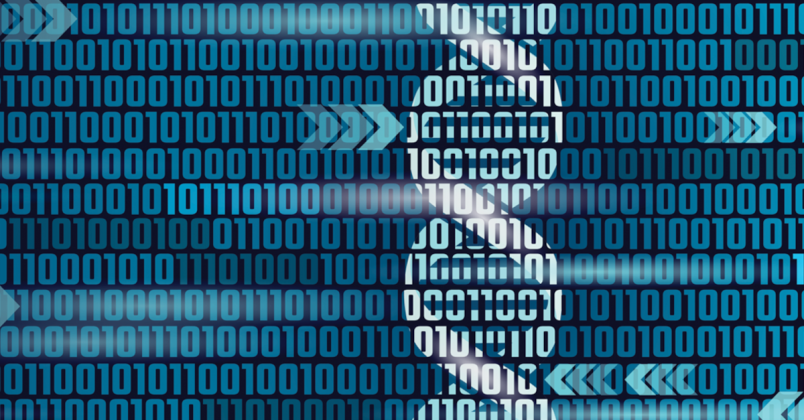
From Biological Inspiration to AI-Driven Algorithms and Back: Modeling, Simulation, and Optimization of Complex Systems
Leonardo specializes in AI-driven modeling, simulation, and optimization of complex systems. His expertise includes co-simulation frameworks, reinforcement learning, formal languages, and computational biology, with applications in synthetic biology, biofabrication, and structural biology. His recent research focuses on advancing AI-based explainability in protein folding.
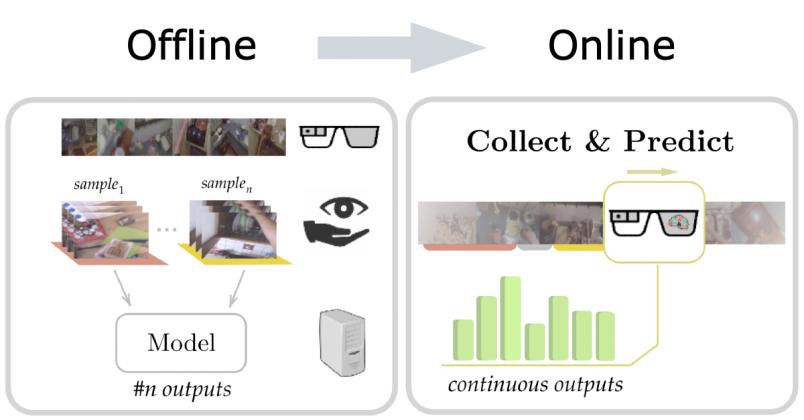
Development of sustainable computer vision algorithms on edge computing devices
Egocentric vision, a specialized computer vision field, analyzes images and videos from wearable cameras. Despite its promising applications in wearable devices, it faces challenges like online processing, environmental bias, and computational cost in real-world settings. My research aims to address these issues to advance egocentric vision for practical use.
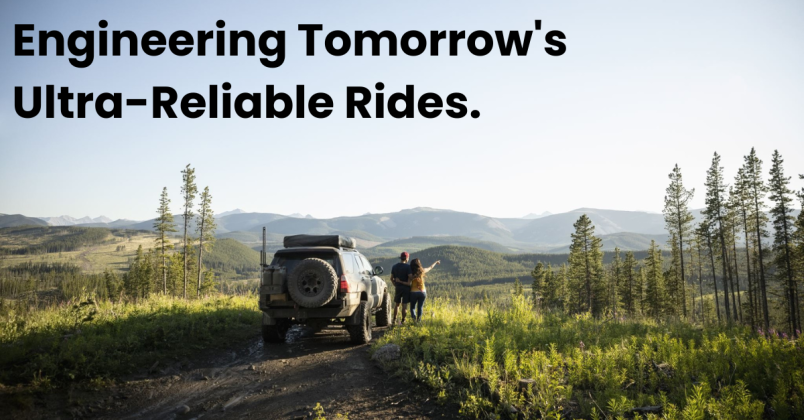
Towards Ultra-Reliable Automotive SoC
Safety-critical devices require testing techniques to ensure their reliability during the entire lifetime. The research aims to integrate and innovate such techniques for modern complex Automotive SoCs, with two goals: decreasing the testing time and costs without losing reliability and collecting on-chip information during the life of the embedded systems to diagnose faulty behaviors of field returns.
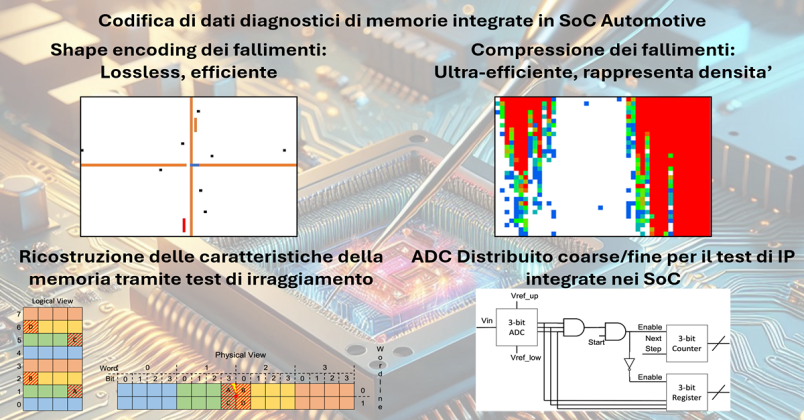
Test and diagnosis of memories embedded in Automotive SoC
My research focuses on the reliability of Automotive SoCs. In particular, I have created a series of algorithms for the compaction and compression of diagnostic data generated during the SoC memory test. I have also developed a method to reconstruct the memory characteristics starting from the irradiation test data and a new type of test-oriented ADC converter.
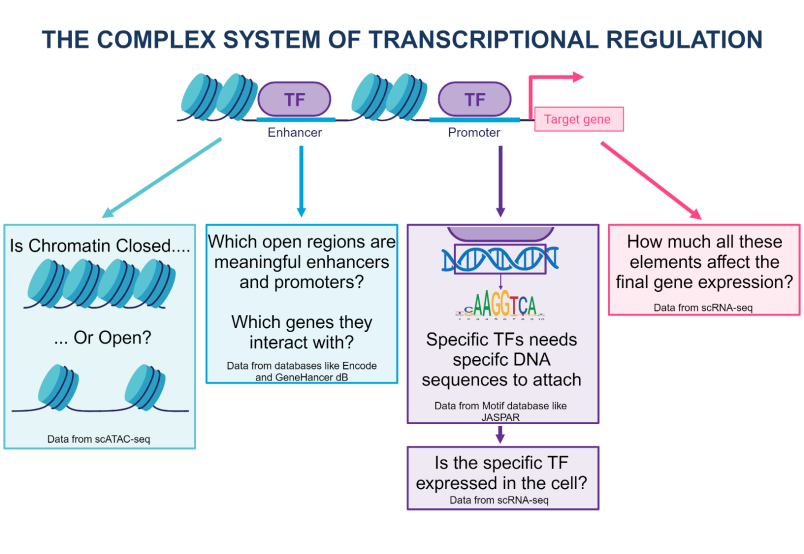
Complex systems science and brain dynamics
Many people may remember the phrase “DNA is the blueprint of life.” But if all human cells share the same blueprint, where does the incredible cell diversity come from? The answer lies in specific gene expression profiles and the complex system of transcriptional regulation. My work focuses on creating computational tools and bioinformatic workflows to investigate and integrate cutting-edge experimental data to shed light on brain dynamics process and cancer research.
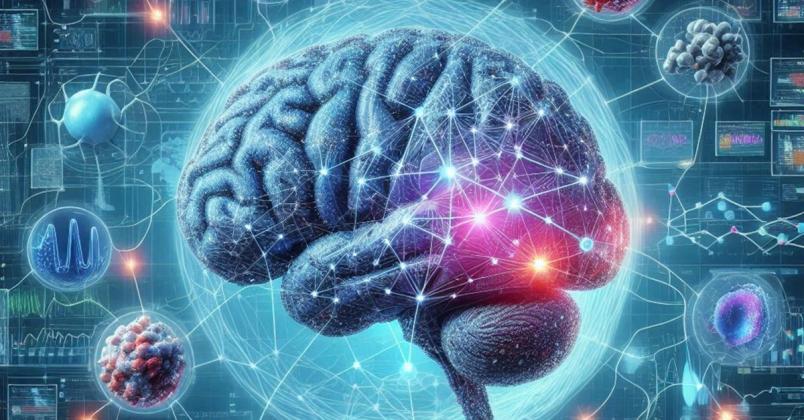
Theory-inspired deep learning
My research combines the benefits of deep learning, which is generalizable but data-intensive, with theory-based models relying on prior knowledge but limited in some real-world scenarios. This involves using various training strategies and enhancing traditional architectures. The goal is to improve efficiency and model convergence speed, creating more expressive tools to explain model outcomes.
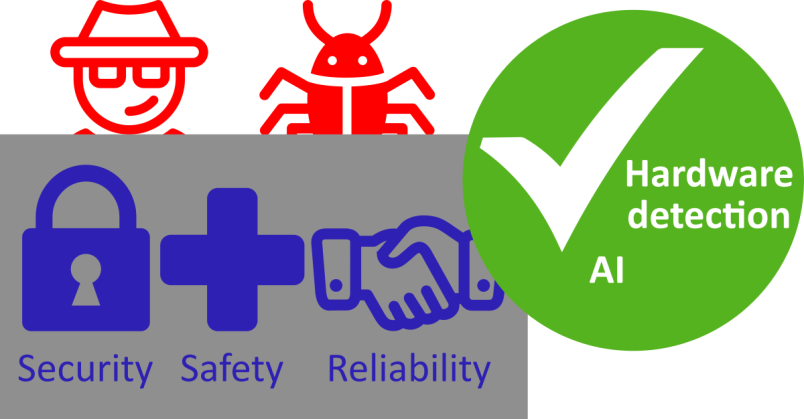
Hardware Based Neuromorphic Resilience to Faults and Attacks
Code anomalies may affect modern computing systems’ safety, reliability, and security. They include bugs, malicious code, and indirect effects caused by faults in the physical layer. This Ph.D. project aims to investigate the capabilities of hardware events and artificial intelligence to improve resilience to code anomalies. This approach has advantages like real-time detection and minimal performance overhead.
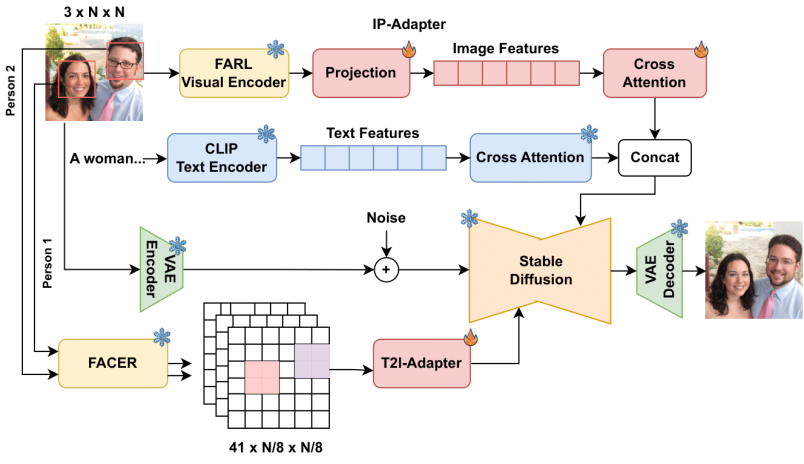
Advanced learning strategies for visual understanding
Developing more fair and robust deep neural networks is my doctoral challenge. By integrating prior knowledge, I guide the learning of DNNs to overcome several key obstacles: limited labeled data, bias, shortcuts, and increase their controllability. My projects include a latent diffusion model-based system for anonymizing images, preserving the original content but removing identifying information.
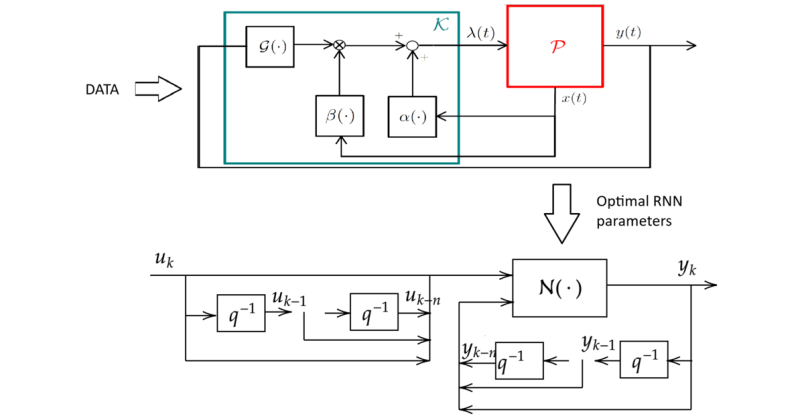
Optimization methods for dynamical system learning
Recurrent neural networks (RNNs) are a popular tool for learning dynamical systems from data. However, training RNNs is widely recognized as challenging due to vanishing gradient issues. To train RNNs effectively, we investigate novel problem formulations and optimization algorithms. To achieve this, we propose a novel paradigm for constrained optimization based on feedback control theory.
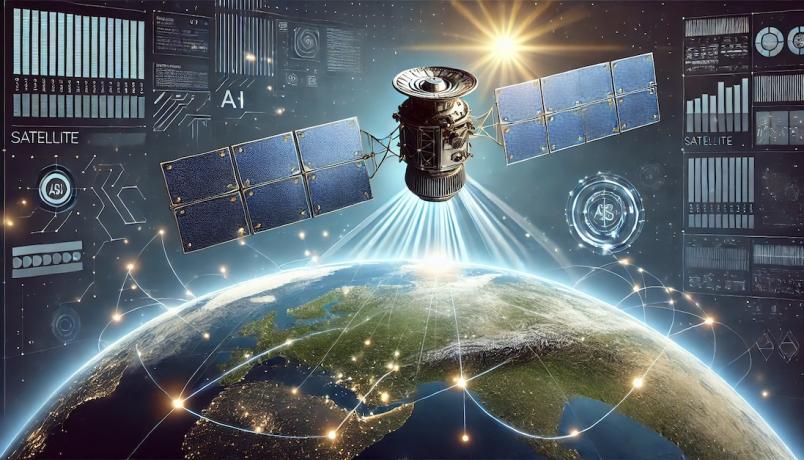
AI-oriented Design Methods for Intelligent Aerospace Computing Systems
Automatization and AI in satellite computing are revolutionizing modern technology, allowing satellites to make autonomous decisions without ground control, greatly benefiting space missions. However, AI's use in space is limited due to unknowns about its behavior in harsh environments. My research focuses on AI algorithm pipelines in space, aiming to develop CAD methods for designing resilient AI algorithms and effectively mapping them onto modern edge reconfigurable devices.
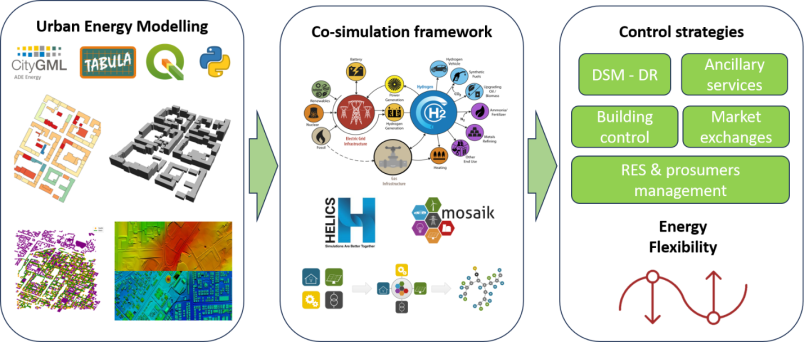
Simulation and Modelling of Energy Demand Flexibility in energy communities
My research aims to explore solutions in the energy sector to enhance flexibility and operational efficiency. Specifically, I began by automating methods for modeling multi-energy systems, followed by creating a standardized co-simulation framework for their simulation. Finally, I tested various machine learning-based strategies to optimize their control. These efforts contribute to advancing the integration and management of complex energy systems.
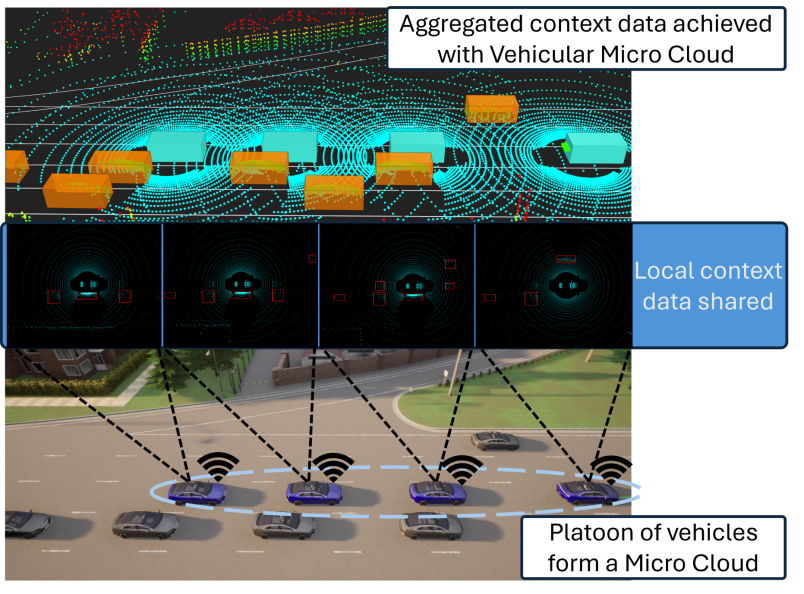
Vehicular Micro-Cloud Architectures and Applications
Future Connected Autonomous Vehicles (CAVs) generate and share vast amounts of data. Efficient sharing and processing of redundant data created by neighboring CAVs is crucial. Vehicular Micro Clouds (VMCs), where clusters of vehicles (such as platoons) collaborate via vehicular networks, offer a promising solution. This research seeks new methods to efficiently aggregate context data within VMCs.
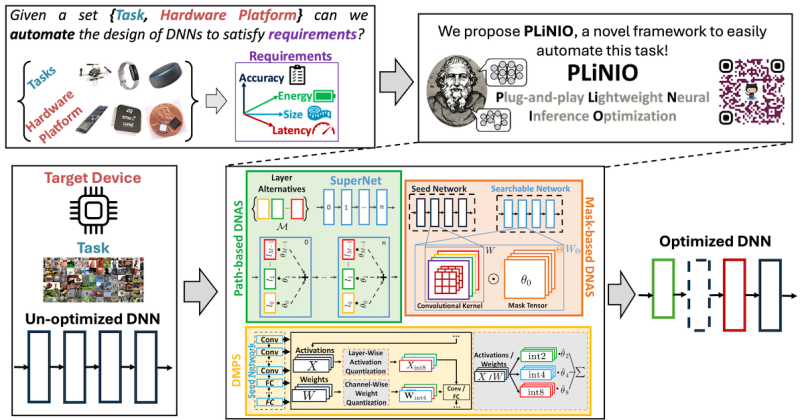
Automatic Hardware-aware Design and Optimization of Deep Learning Models
Deep Neural Networks (DNNs) popularity requires optimizing them for many use-cases, especially for edge devices with limited resources. Manual tuning is suboptimal, leading to Neural Architecture Search (NAS). However, NAS has limitations in complexity and hardware awareness. This thesis addresses these issues with PLiNIO, an open-source framework for efficient, hardware-aware DNN optimization.
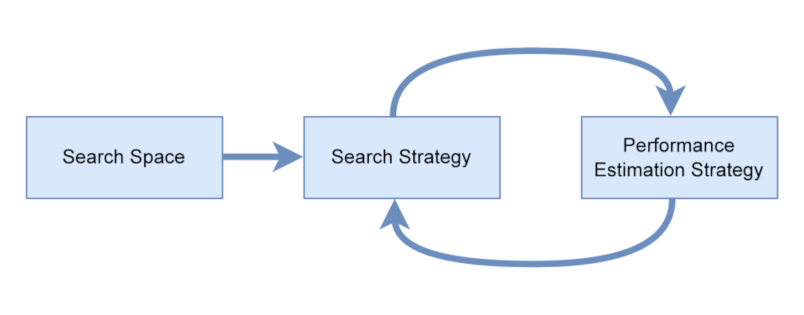
Hardware-oriented Neural Architecture Search
In the last few year, Neural Architecture Search (NAS) algorithms have emerged to automate neural network design. This project aims to solve this issue by developing scalable NAS methods able to satisfy hardware-related requirements and ease the development and deployment of efficient deep learning models for real-world applications, from embedded devices used in robotics to large-scale clusters.
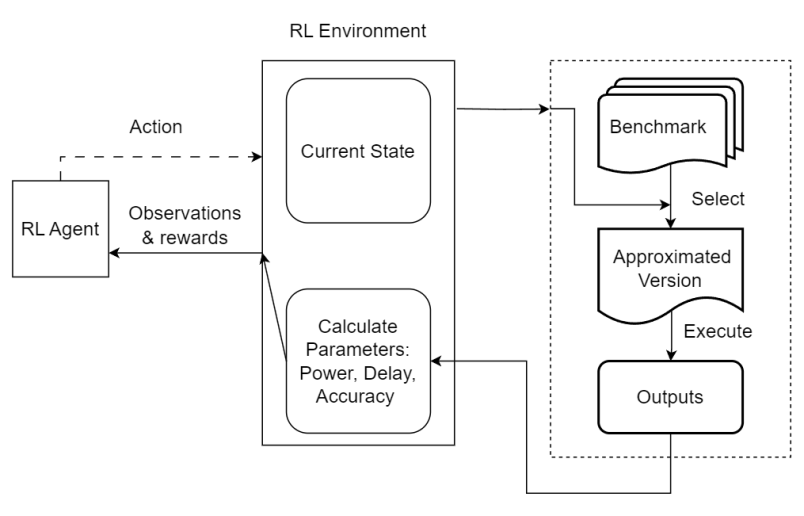
Fast and Accurate Prediction of the Impact of Approximate Operators on a Complex Computation
Approximate Computing (AxC) techniques trade off accuracy for performance and energy efficiency. To address the problem of finding optimal AxC techniques for each application, we proposed 2 Design Space Exploration approaches: 1- an Interval Arithmetic-based approach to rapidly evaluate the approximation error. 2- a Reinforcement Learning approach to efficiently explore a vast design space.
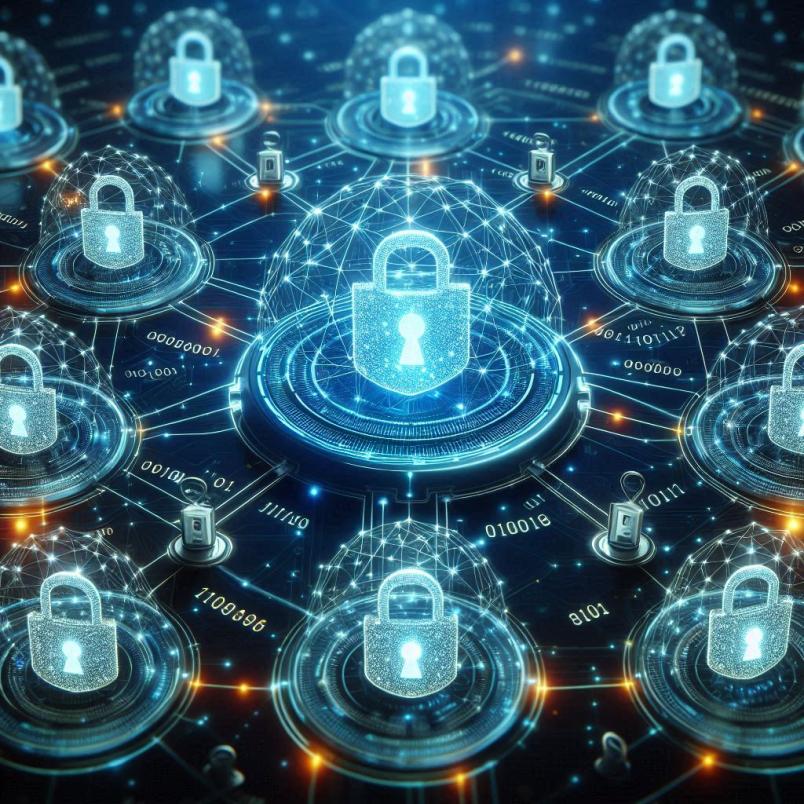
Zero-Trust Security of Network Nodes
My research focuses on defining Zero-Trust architectures to secure modern ICT infrastructures, which now involve distributed data processing across edge, fog, and cloud computing. I explore techniques for device identity verification, secure node configuration, and verification of the security posture throughout the entire lifecycle of each component, ensuring robust security in dynamic environments.
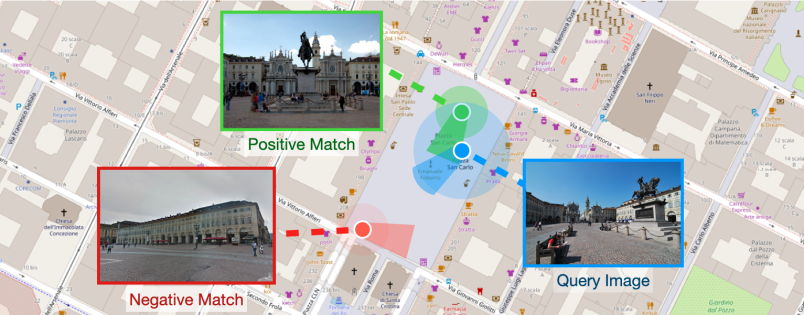
Attention guided cross domain geolocalization
Geolocation is a challenging task as photos may offer few cues about their location. Traditional algorithms rely on the trained features The problem is challenging when there is a shift between training and test data distribution. My research studies the problem of geo-localization across visual domains. We combine attention mechanisms with modern domain adaptation algorithms.
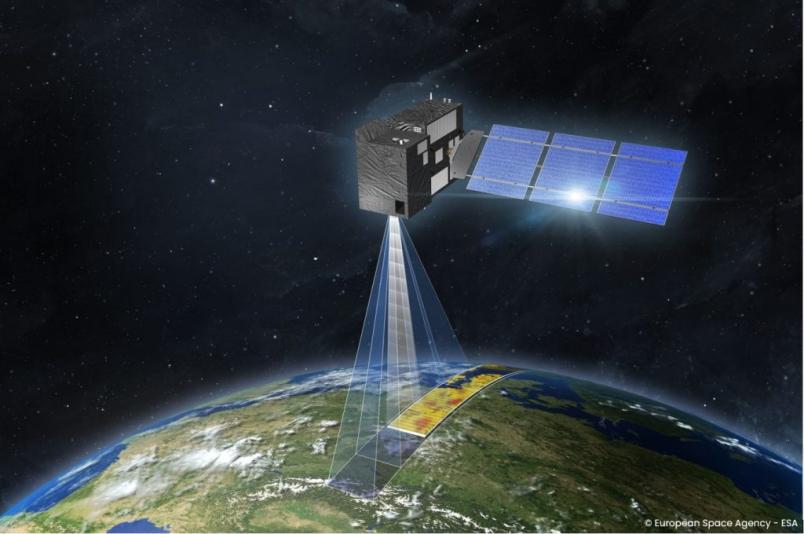
Multi-Test and Validation Platform for AI-oriented Sensor System Integration
AI reliability is crucial for safety-critical application. My research focuses on developing reliable AI accelerators. Specifically, I designed zero-overhead self-test methods and advanced algorithm-based fault mitigation. My work has been applied in industry, including the Zodiac Pioneer Space Mission and the TERRAC project with the European Space Agency.
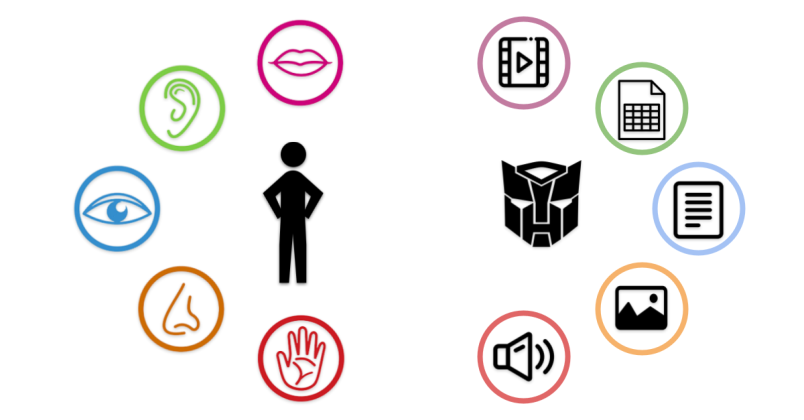
Exploring the use of deep models in multimodal scenarios
My PhD research focuses on advancing multimodal learning, particularly in vision-language tasks. I analyze social media content to detect hate speech and fake news, and work on Visually Rich Document Understanding through VQA and key information extraction. Additionally, I explore multimodal tasks involving audio, such as podcast summarization and video emotion recognition.
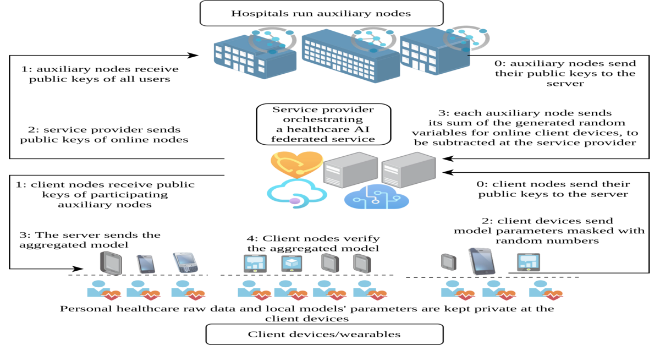
Enhancing Security and Privacy in Federated Learning through Advanced Adversarial Analysis
Federated learning has emerged as a promising approach for preserving privacy. However, recent studies show that private training data can be leaked through many attacks. Our research examines these security weaknesses, focusing on how adversaries extract private information from gradients, with the goal of developing comprehensive solutions to protect data privacy in federated learning.
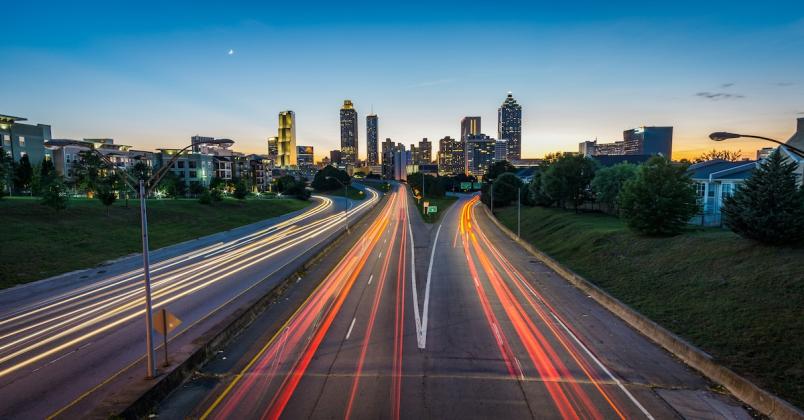
Exploring AI solutions to unveil Urban Data
The future of smart cities relies on the combination of urban data science and Artificial Intelligence, which are crucial for enhancing citizens' quality of life and integrating new technologies. This research exploits advanced machine learning and deep learning techniques to transform complex and heterogeneous data into actionable insights, providing effective solutions to real-world challenges.
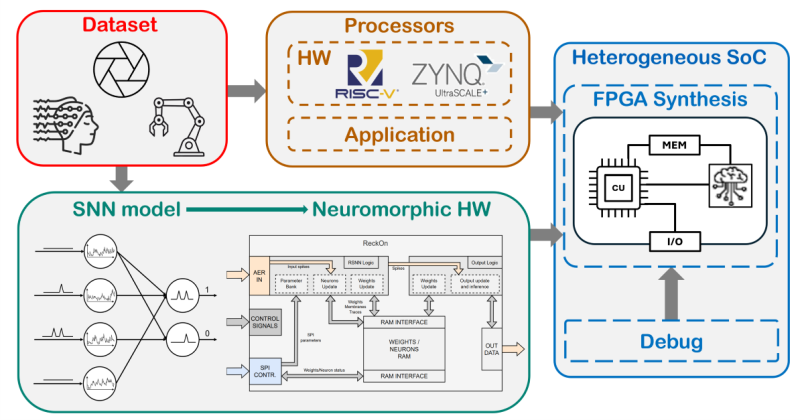
Design of digital/neuromorphic computing systems and SDK
This PhD research, funded by EBRAINS-Italy, aims to accelerate the engineering process behind the simulation of Spiking Neural Networks through the creation of heterogeneous SoCs on FPGA-based systems. The system will support the development of neuromorphic applications by combining the advanced computing capabilities of SNNs accelerators and the configurability provided by traditional processors.
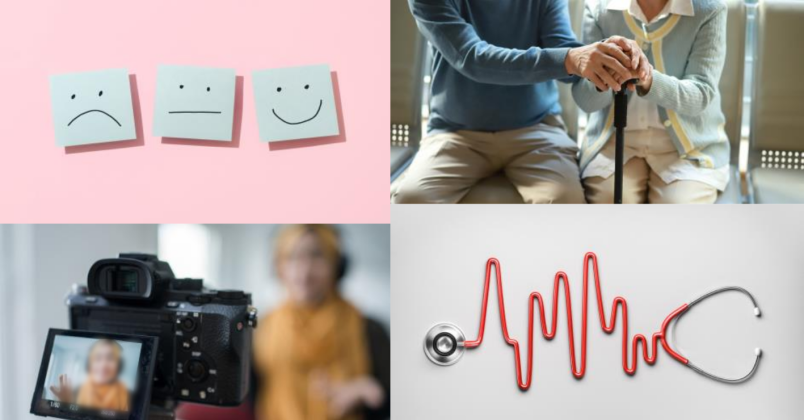
Early markers to predict the evolution from Mild Cognitive Impairment to Overt Dementia, and support to differential diagnosis of diverse forms of dementia
Dementia is a decline in cognitive function and the leading cause of disability in the elderly. Early diagnosis is challenging but crucial to improve quality of life. This work investigates facial expressions as possible early markers of dementia. Artificial intelligence methods for facial expression analysis are explored to support early and differential diagnosis of different types of dementia.
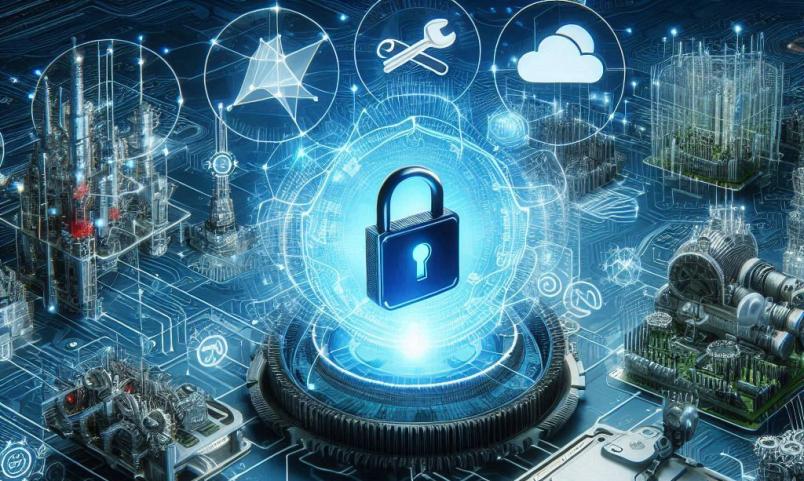
Security of cyber-physical systems
My research focuses on securing devices by designing and implementing solutions leveraging principles like Trusted Computing. This involves evaluating hardware and software support for trusted execution on limited-capability nodes. The goal is to demonstrate a secure CPS ecosystem with strong identity, secure communications, and integrity monitoring using Trusted Computing and AI techniques.
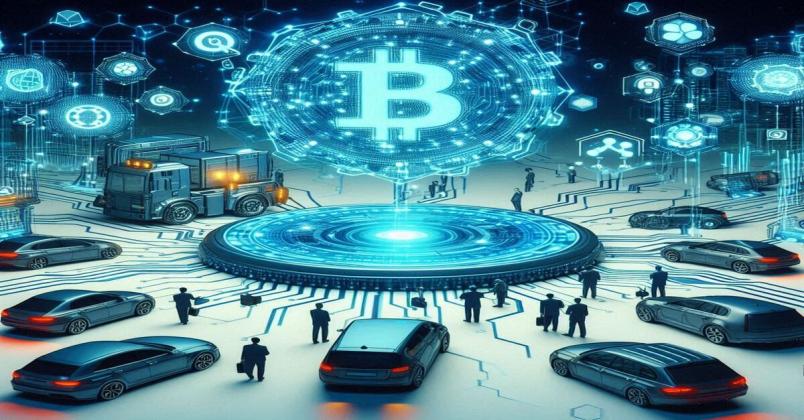
Blockchain Technology for Intelligent Vehicles
As a second-year PhD student, I am advancing blockchain technology for smart vehicles. My research develops innovative distributed ledger solutions to enhance vehicle data traceability, autonomous system integration, and cross-platform interoperability. By leveraging cutting-edge blockchain protocols, I aim to revolutionize smart transportation addressing key challenges for intelligent vehicles.
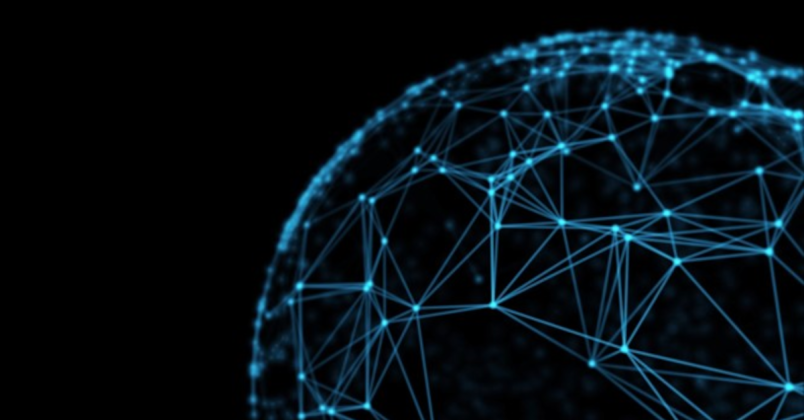
GPU-based Parallel Techniques for the Evaluation of Reliability Measures of Large System-on-Chip devices
Graphs can be used to represent almost any problem and are therefore used in a multitude of fields. This research focuses on understanding the intricacies of hard graph problems and the main parallelization schemes applied to them. These techniques will be applied to the implementation of a parallel logic simulator that is able to exploit the power of many-core architectures.
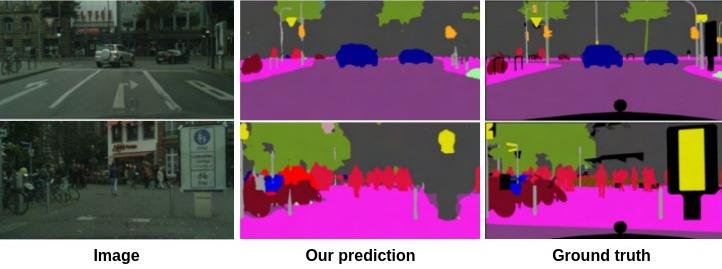
Enabling complex computer vision tasks on the edge: applications to semantic segmentation and beyond
Understanding scenes is crucial for intelligent systems to interpret and interact with their environments. This encompasses tasks like image segmentation (classifying pixels into categories) and referring segmentation (identifying objects from textual descriptions). My research focuses on developing robust models to handle real-world scene complexity, emphasizing efficient, real-time solutions.
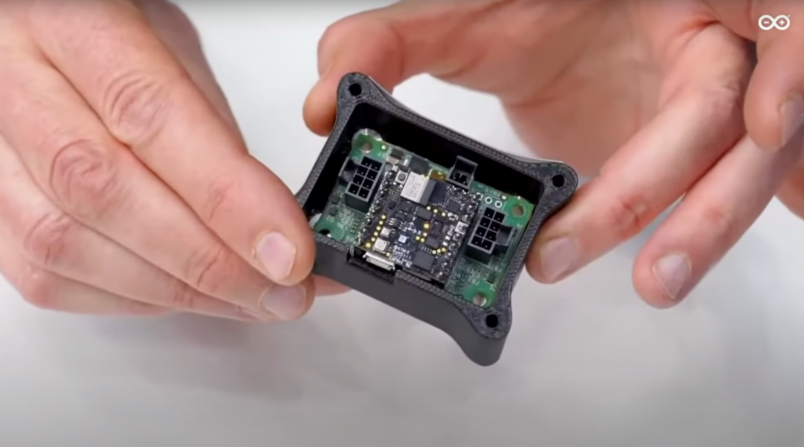
Innovative solutions for Industrial IoT
The goal of the research is to develop solutions to apply the concept of Industrial Internet of Things (IIoT) to machinery in the beverage and food industry. I analyze industrial needs to define the challenges and problems to be solved. I collaborate with AROL to understand the requirements of the bottling sector and define a reference architecture for an IIoT solution that includes field, edge, cloud, and connectivity, with a focus on functionalities such as storage and processing.
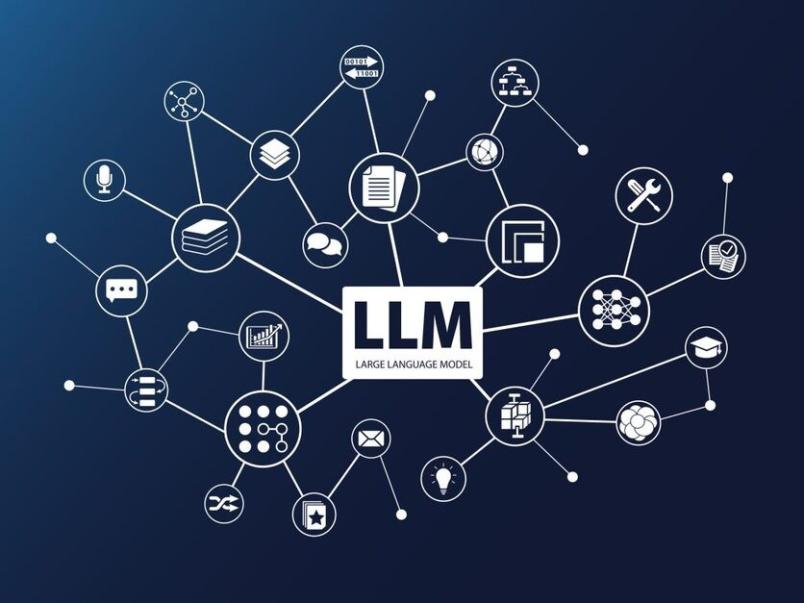
Explainable Large Language Models
Large Language Models (LLMs) excel in text understanding and generation. However, their complexity makes it difficult to understand the decision path they took to make a prediction or to generate text. Therefore, it is crucial to develop tools that can reveal model behaviour, ensuring more reliable LLMs.
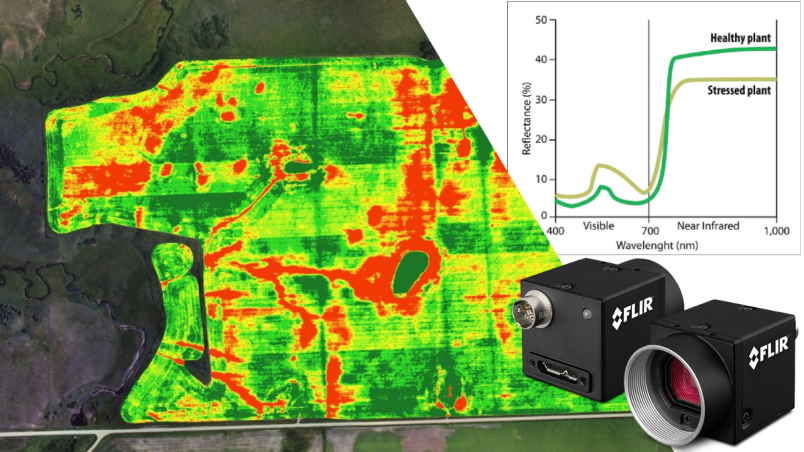
Analysis of Multispectral Plant Imaging Data
Multispectral imagery can revolutionize disease prediction methods and plant phenotyping in agriculture. My research aims to identify the most effective wavelengths, improving prediction accuracy and simplifying the classification process by reducing the number of variables used. This approach will lead to developing more cost-effective and efficient tools for these applications.
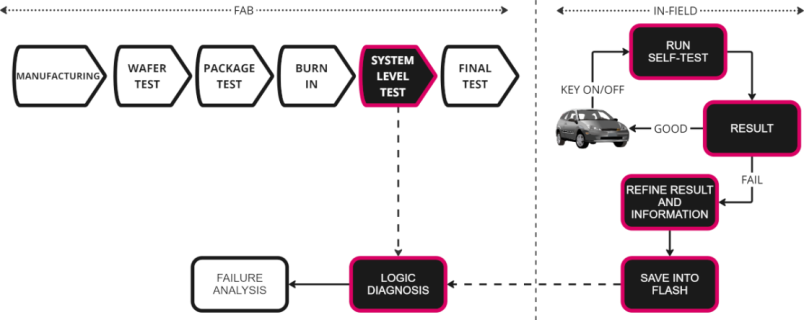
Manufacturing and in-field testing techniques
This research aims to enhance the reliability of modern SoC in automotive and safety-critical sectors. Current testing methods fall short in ensuring reliability, necessitating new approaches covering a device's lifecycle. Key areas include improving functional manufacturing testing to reduce yield loss, developing in-field self-test routines for high fault coverage, and using logic diagnosis.
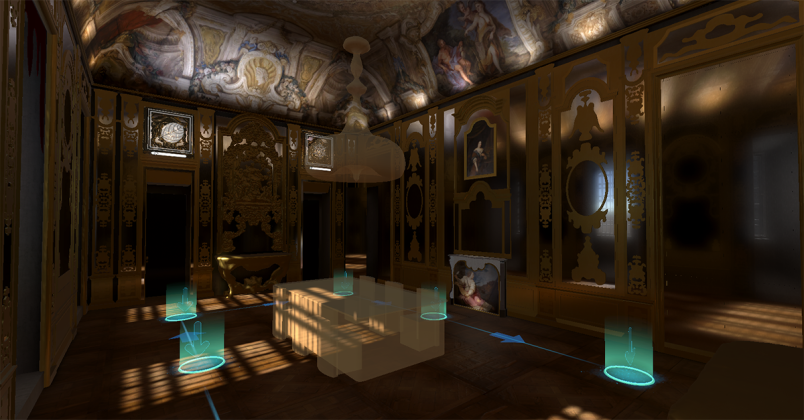
XR for Cultural Heritage Accessibility
The topic of eXtended Reality (XR) for Cultural Heritage (CH) is highly relevant in the scientific community, particularly in computer science. The number of works combining CH and XR has surged, covering virtual exhibitions, reconstructions, narrative experiences, and educational systems. Accessibility in XR and CH is either implicitly or explicitly addressed, but deeper exploration is needed. Key elements are physical and perceptual accessibility, catering to users with disabilities or neurological disorders.
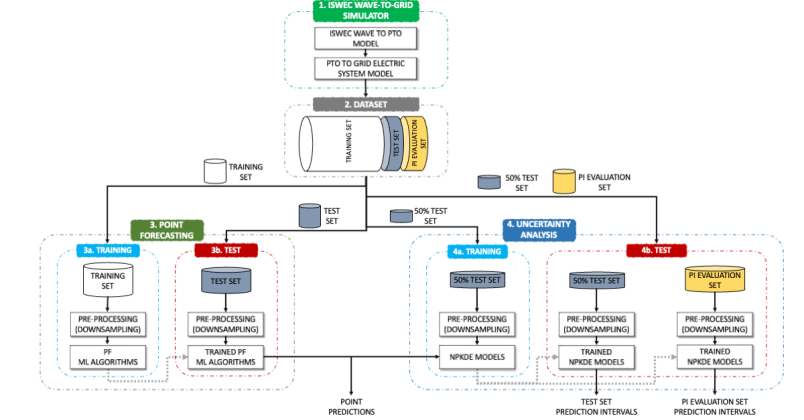
Digital Twin Models for Marine Offshore Renewable
The PhD activities focus on the design and development of Digital Twin of real-world Wave Energy Converters (WECs) to unlock algorithms both i) to forecast energy production in the short and mid-term and ii) to promote predictive maintenance strategies. The objectives also comprise the development of an Internet of Things (IoT) platform able to collect, store and retrieve the WEC’s sensed data.
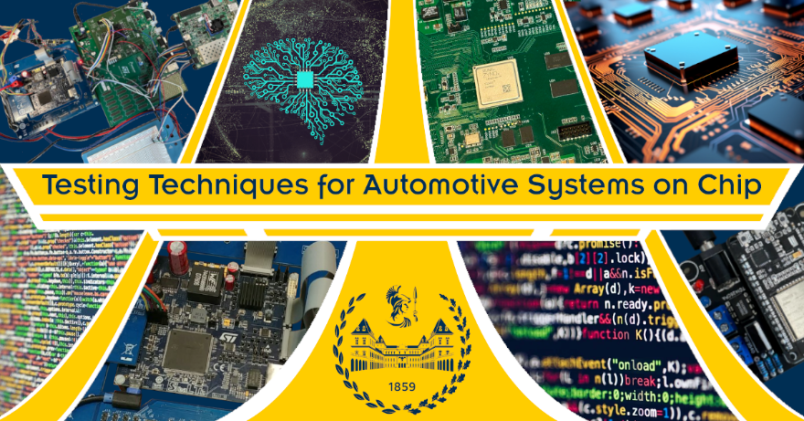
Testing Techniques for Automotive Systems on Chip
The proposed research project will focus on testing techniques used for automotive systems-on-chip. The study aims to identify the most effective testing methods to recognize chip defects and reduce the number of defective microelectronic devices entering the market. The outcomes of this study will contribute to the creation of new testing techniques that can be applied to microelectronic devices.
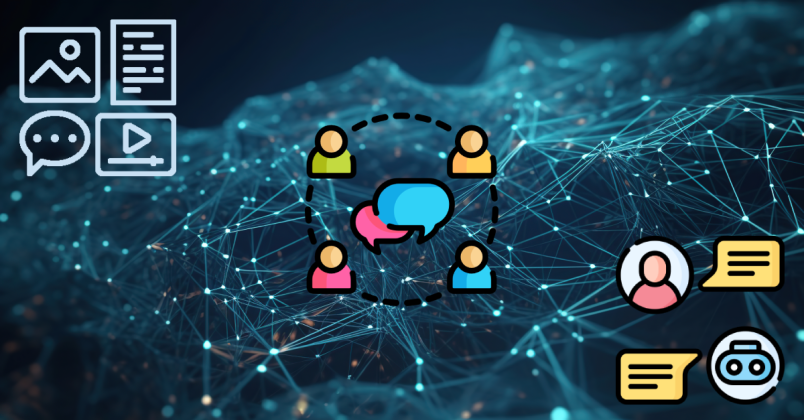
Conversational AI – New tasks and models for conversational applications
Given the rapid proliferation of Conversational AI across academia and industry and its widespread user adoption, my research aims to explore the diverse tasks and models within this field. By leveraging the unique characteristics of conversational data, my goal is to develop more effective solutions for both new applications and traditional NLP tasks applied to the conversational domain.
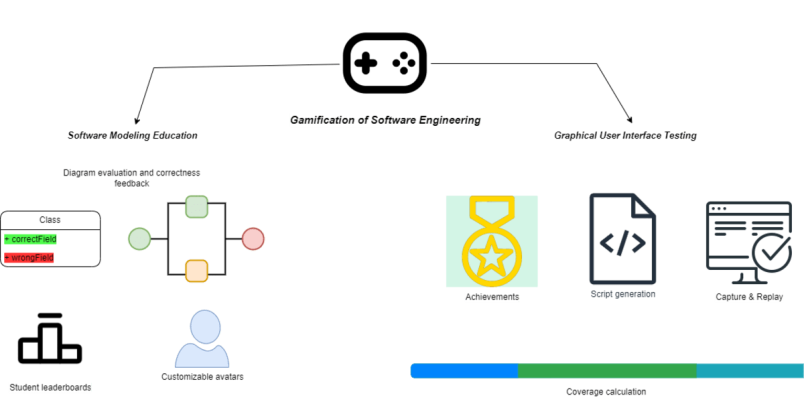
Advancing UI testing techniques
My PhD research focuses on adopting gamification (using game-like elements in non-recreational concepts for increased enjoyment) to improve two software engineering topics: requirements definition through software modeling, and user interface testing. For the former, I have defined a framework for gamifying modeling education, and for the latter I have developed IDE and web plugins for UI testing.
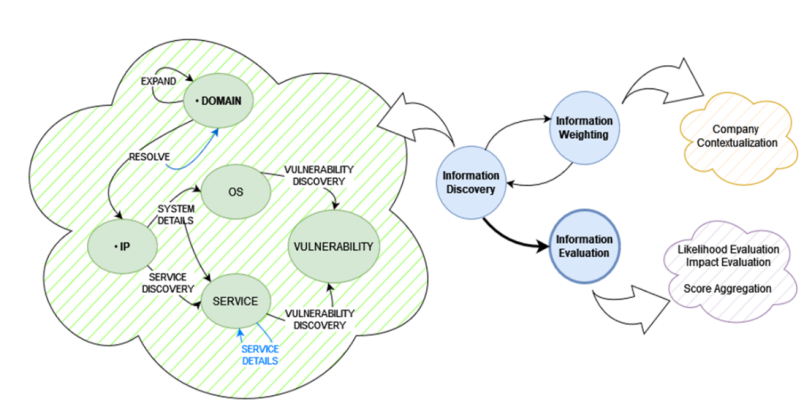
Automatic Analysis of Cybersecurity Risks
The research aims at developing methodologies for the collection and evaluation of cyber risk related information so that companies with low technical skills and resources can understand their exposure to cyber threats. The research started with the development of an automated system that can collect publicly available information, assign it a score and aggregate these evaluations into summarizing indexes for ease of comprehension. The current focus is on the validation of the scoring system and the exploration of how AI can support this process.
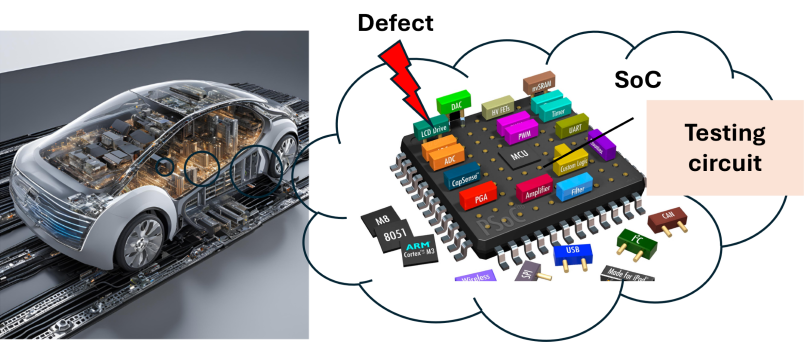
Functional Safety Techniques for Automotive-oriented Systems-on-Chip
With recent improvements in System-on-Chip (SoC) technologies, the complexity of SoCs is advancing, and their dimensions are shrinking, so they have more frequent physical defects. Automotive SoCs are used in fields such as electric vehicles, aerospace, etc.; testing is needed for high application quality. The main target of my research is to develop self-testing circuits inside SoCs.
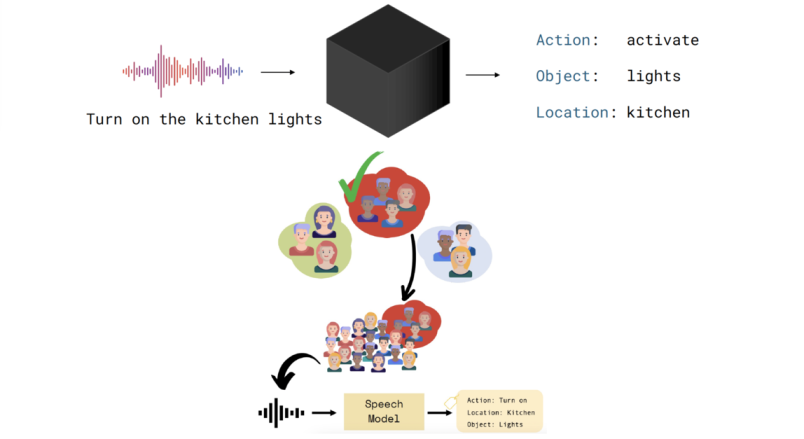
Explainable AI for spoken language understanding
End-to-end spoken language understanding (SLU) models infer semantic information from spoken signals, improving accuracy over traditional SLU models. Their black-box nature lacks in explainability and transparency. Collaborating with Amazon AGI, my research focuses on explaining SLU model prediction, identifying subgroups with anomalous behavior, and enhancing performance while mitigating biases.
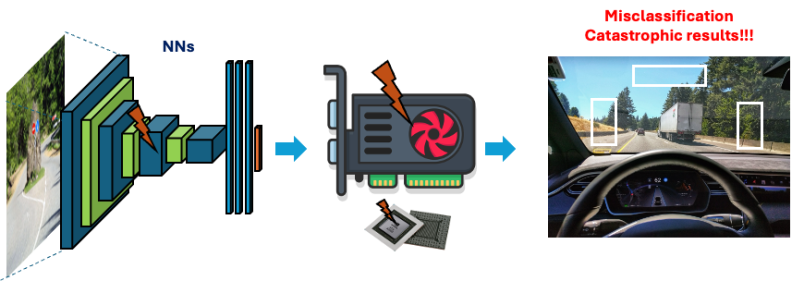
New Techniques for the Reliability Evaluation of Neural Networks running on GPUs
The reliability of neural networks deployed on GPUs is crucial in many domains (automotive). Given the complexity of both hardware and software, estimating reliability figures is challenging. My activities focus on new approaches to characterize the vulnerability to faults affecting the hardware while exploring methods to detect faults and developing clever strategies to enhance system resilience.
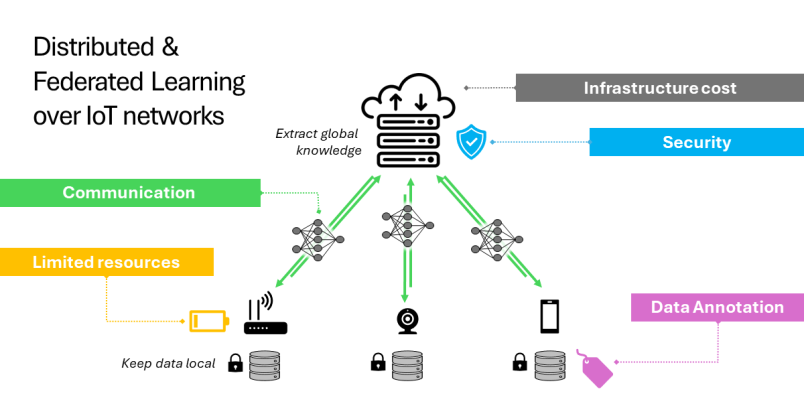
Distributed and Federated Learning over IoT networks
Distributed and Federated Learning enable the sharing of data-driven knowledge among smart sensors under privacy and infrastructure restrictions. Yet, communication bottlenecks and limited device resources challenge the development of scalable and robust distributed learning. Novel and multifaceted solutions are required to efficiently address infrastructure costs, data annotation, and security concerns to unlock the potential of edge intelligence.
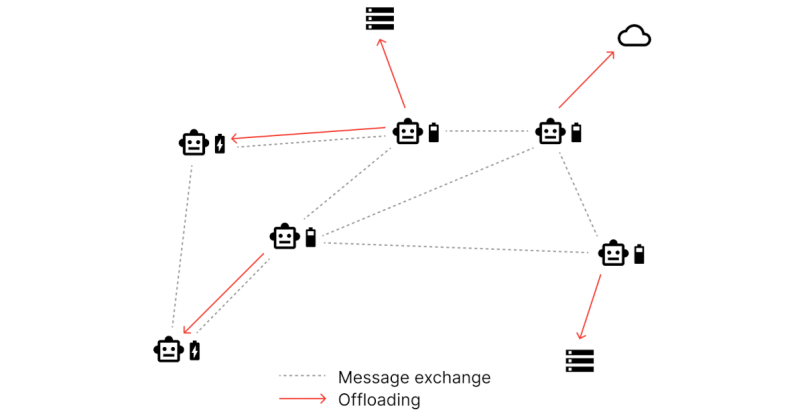
Efficient 6G Softwarized Network Infrastructures
My research enhances Kubernetes clusters for seamless resource sharing in the Computing Continuum, offloading tasks from robots to 6G edge or cloud resources. This reduces battery use and boosts computational power by accessing advanced resources like GPUs, and idle charging robots to improve efficiency, aiming to create a more efficient computing environment for autonomous robots.
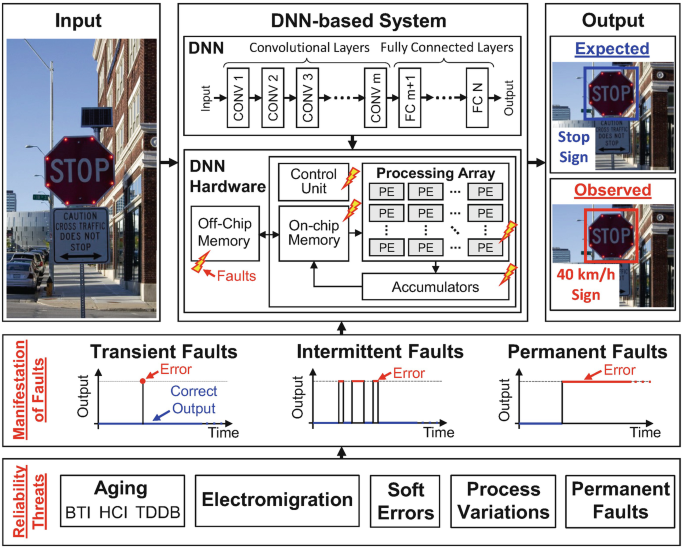
Robust, accurate and efficient deep neural networks for satellite and mobility applications
Computing platforms in vehicles and satellites are becoming more powerful. However, deploying deep learning (DL) applications on these platforms requires deep neural networks (DNNs) that maintain high accuracy, efficiency, and robustness, even in the presence of transient faults like radiation-induced errors. This research focuses on ensuring DNNs meet these stringent standards.
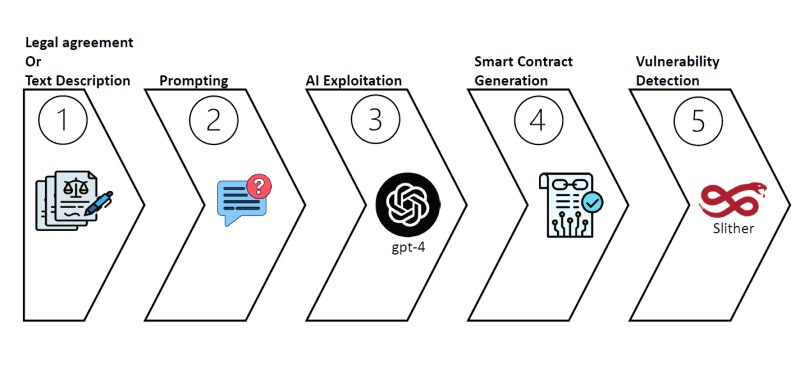
Analysis, search, development, and reuse of smart contracts
My research in blockchain technology focuses on innovative approaches to simplify smart contracts creation. By leveraging Large Language Models and advanced visualization techniques, the objective is to make the complex process of writing smart contracts more accessible and efficient. This innovative approach combines cutting-edge AI with visual representations to enhance blockchain development.
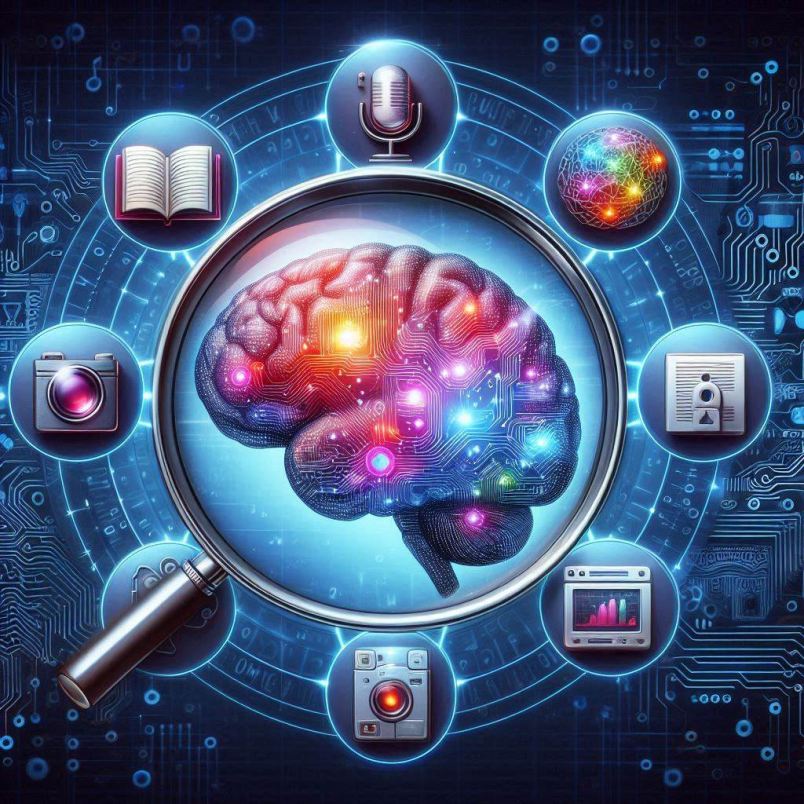
Neural Explainers and Multimodal Learning
My PhD research focuses on developing and exploring new neural approaches to enhance the interpretability of complex AI models and advancing multimodal learning techniques to effectively integrate data from diverse sources, such as text, images, and tabular data. By achieving this, I aim to design AI systems able to make existing AI models more transparent and explainable, providing deeper insights into their decision-making processes and fostering greater trust in their applications across various domains
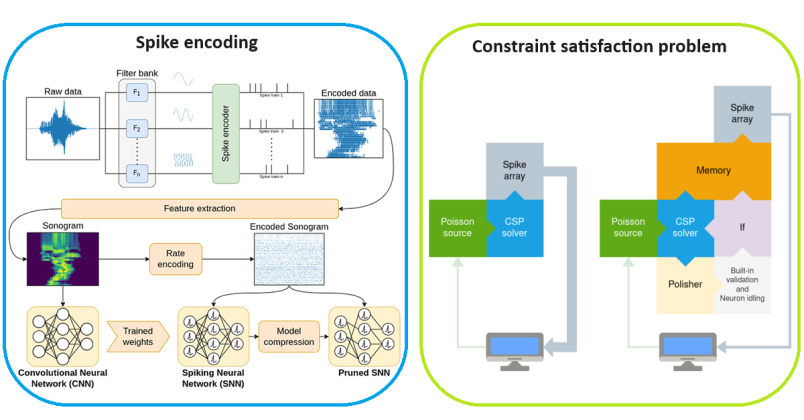
New computational paradigms for neuromorphic HW
The aim of the PhD research activities is to investigate the novel computational paradigm enabled by neuromorphic models, implemented with spiking neural networks, in the areas of machine learning and edge computing. The focus is on digital-to-spike data encoding methods and the adoption of these networks to solve problems belonging to the class of constraint satisfaction problems.
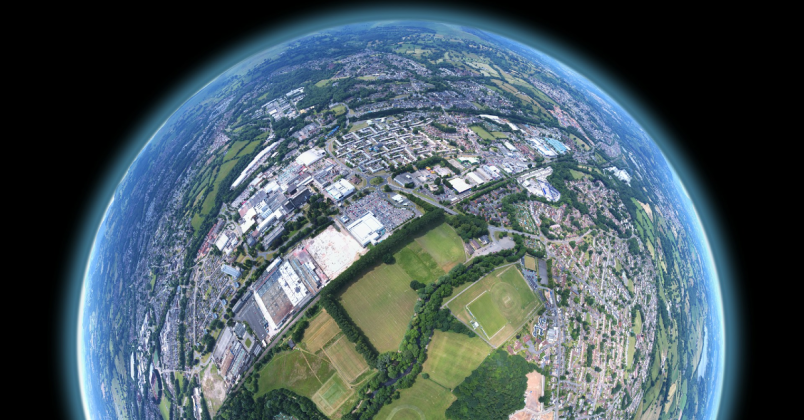
Efficient and Effective Deep Learning for Crisis Management and Ecology
The recent availability of public satellite imagery and textual information are excellent sources to support ecological transition and crisis management. The research focuses on developing efficient and scalable machine-learning solutions to help humanity face great challenges, such as managing natural disasters, preserving green areas, and improving food production.
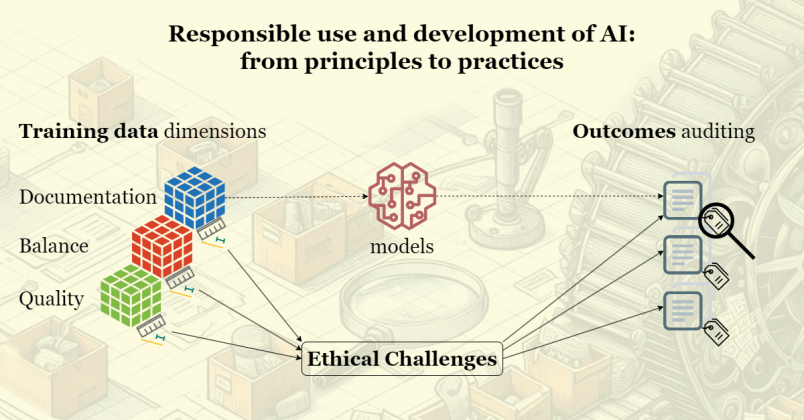
Responsible development and use of AI: from principles to practices
I am on a journey to promote the responsible development and use of AI. My research focuses on creating a metrics-based framework for empirical evaluation of training data. By looking and data quality, balance and documentation, I aim to address ethical challenges in AI. I'm also auditing the outcomes of AI systems to investigate the most common patterns of discrimination.
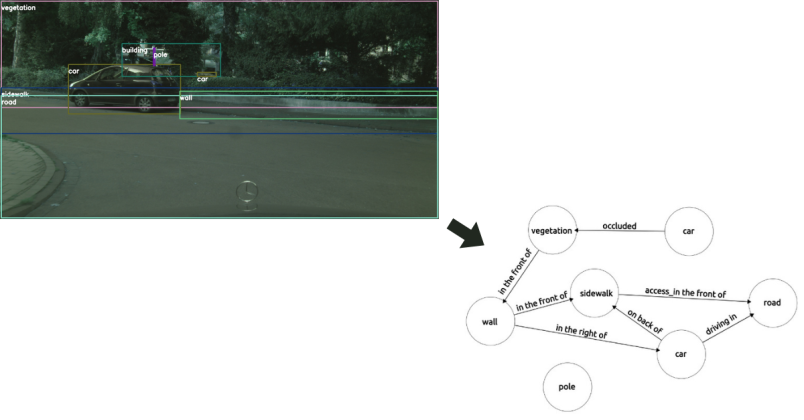
Neural-symbolic scene integration & generation
Neuro-Symbolic (NeSy) techniques enhance scene understanding by combining neural learning with symbolic logic. As a second-year PhD student, my research focuses on using NeSy in Scene Graph Generation (SGG) to detect objects and refine relationship predictions in visual scenes. Applications include autonomous driving and improved human-computer interaction, combining robustness and clarity.
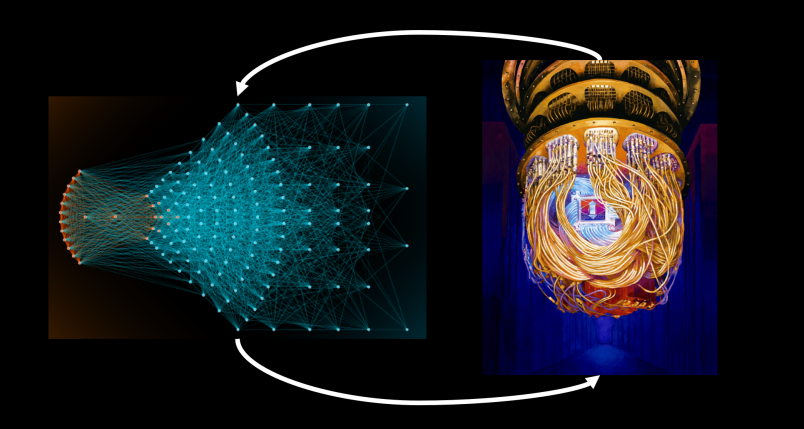
Quantum Machine Learning
QML explores the applications of quantum computing to machine learning and vice versa. It involves both using quantum algorithms to enhance traditional ML tasks, potentially offering exponential speedups, and applying ML techniques to quantum control, improving the efficiency and performance of quantum operations at the physical level.
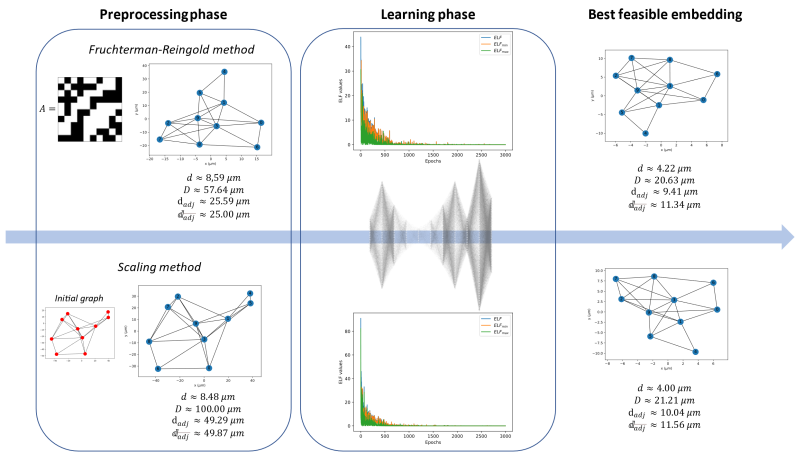
Quantum Machine Learning Applications and Algorithms for Industrial Use Cases
Quantum computing faces challenges such as low qubit counts and limited coherence times, hindering the use of disruptive algorithms. To align the technology with practical industrial applications, I conduct research in hybrid quantum-classical algorithms, specifically focusing on quantum optimization and quantum machine learning on NISQ (Noisy Intermediate-Scale Quantum) devices.
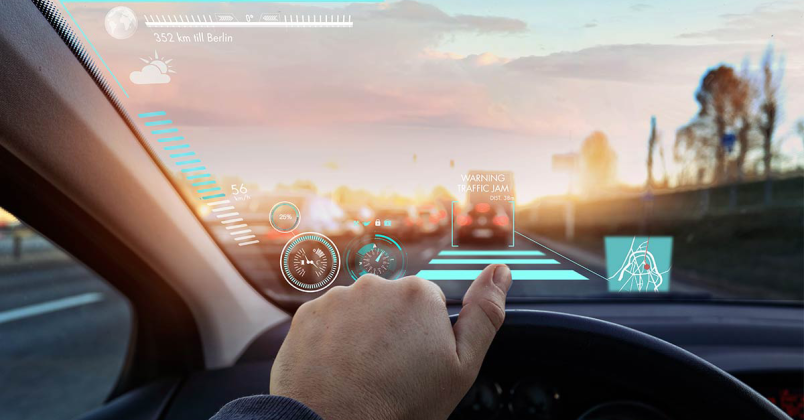
In Car AR HUD
My phd focuses on Augmented Reality on Head-up Displays for automotive in collaboration with Reply, funded by a PNRR scholarship. Using the CARLA driving simulator, I investigate the role of AR HUDs in realistic scenarios. Using such a tool result in a flexible, shareable, and cost-effective approach, creating shareable and innovative solutions for a safer and more intuitive driving experience.
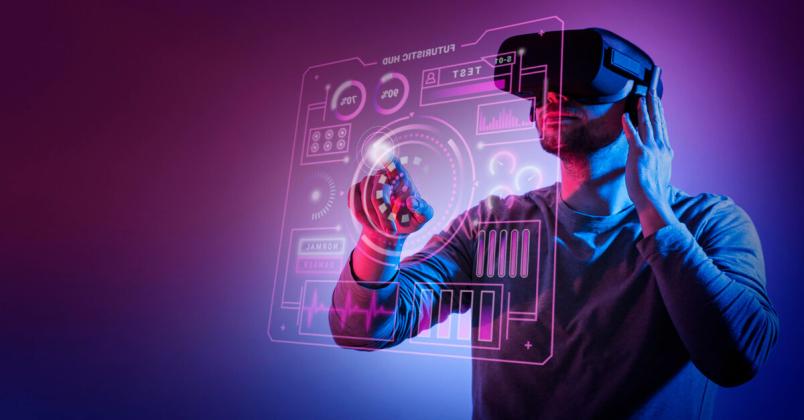
Digital Twin, Presence & Interaction in Metaverses
As a second-year PhD student, my research aims to improve the metaverse, immersive, social and decentralised 3D world. My goal is to enhance user interaction, immersion and presence through realistic representations, intuitive interaction mechanisms, creating authentic virtual experiences and social interactions through cutting-edge technologies and digital twinning solutions.
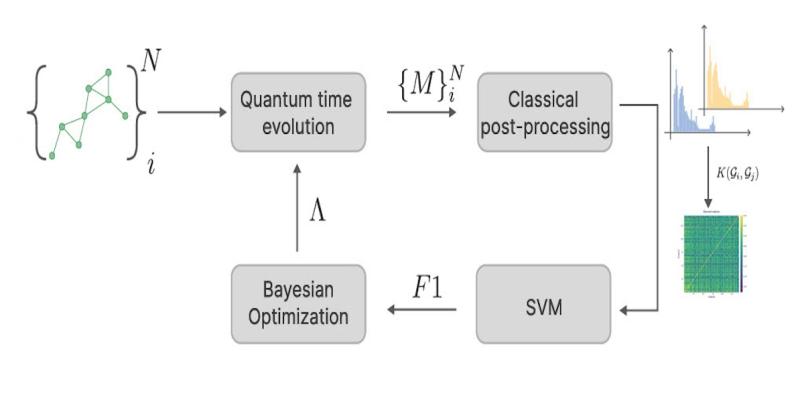
Quantum Machine Learning Applications and Algorithms for Technology Transfer
Quantum Machine Learning holds the potential to outperform classical methods. However, the embryonic stage of these technologies limits the applicability of such algorithms to real-world problems. My work focuses on reducing this gap by matching applications to the most suitable combination of state-of-the-art QML algorithms and technological platform, with a focus on neutral atom simulators.
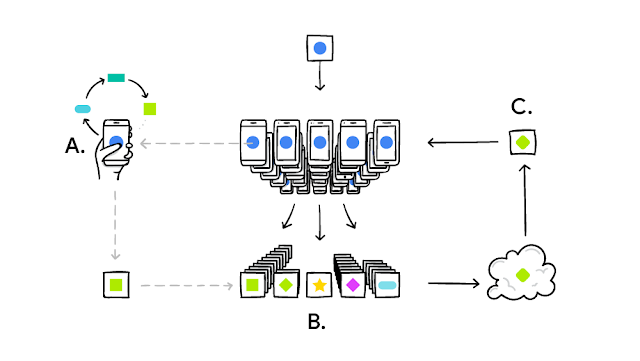
Algorithms and architectures for distributed training of deep neural networks
Modern success of deep learning relies on increasingly complex models, whose training requires large amounts of data and computing resources. This PhD project investigates the problem of distributing the training of deep neural networks and developing novel algorithms and model architectures to enable efficient and effective decentralized training for next generation deep learning applications.