The FAIR principles are guidelines to make all research results such as (but not limited to) research data, software and protocols Findable, Accessible, Interoperable and Reusable. They were formulated and formally published in 2016 in Nature Scientific Data.
FINDABLE
First of all data should be easy to find for both humans and computers and this can be done through rich and detailed metadata [labels describing author, title, date, provenance...] and unique and persistent identifiers [stable URLs to which that resource will forever correspond, a DOI, a handle, etc.].
Moreover, the data must be kept for at least 10 years in an archive that offers guarantees, stored in multiple copies and in a secure environment even during the research, not only when it is finished.
ACCESSIBLE
Data and metadata must be Accessible, which does not mean “open” but knowing how to get to the data and how to possibly download it through an open, free and implementable protocol everywhere. Authentication and authorization procedures and/or confidentiality agreements may be in place.
The guiding principle to be followed is “as open as possible, as closed as necessary” depending on the specific requirements, e.g., for the protection of sensitive data or Intellectual Property.
There may be FAIR data closed for security or privacy reasons.
Metadata again play a key role to signal the need for particular transmission protocols (other than http://) or the presence of API – Application programming Interface.
Ideally, to be Accessible, data should be saved in non-proprietary, uncompressed, unencrypted formats with documented standards.
INTEROPERABLE
Data must be described using standards relevant to the community. A valuable tool in this respect is the FAIR sharing register, a curated and informative resource on data and metadata standards, inter-related to databases and data policies.
REUSABLE
The ultimate goal of the FAIR principles is to optimise the reuse of data. To achieve this, metadata and data should be well-described so that they can be replicated and/or combined in different settings and should have a clear and accessible data usage license, such as the Creative Commons (here the License Selector Tool useful for choosing a licence).
Each letter in the FAIR acronym refers to a list of principles with a total of 15 principles altogether. You can find the complete list and more information at the dedicated GOFAIR webpage.
Checking whether one's research data have been managed according to FAIR principles can be a difficult and laborious task. To help in this task, several tools and metrics have been defined over the years. EUDAT, in particular, has developed a self-assessment checklist, which can easily be translated into a series of questions:
Findable
- Has a persistent identifier (e.g. DOI, Handle, URL) been assigned to the dataset?
- Has the dataset been described with detailed, informative and accurate metadata?
- Is the metadata recorded in an online catalogue or data repository that is indexed in search engines?
- Does the metadata include the persistent identifier assigned to the dataset?
Accessible
- Does the persistent identifier correctly access the data or associated metadata?
- Does the protocol for retrieving data and metadata respect a standardised and recognised language such as that of web pages (HTTP)?
- Will metadata always be public, visible and indexable even if the data are not in open access or are no longer available?
Interoperable
- Is the data available in open formats or at least in documented and disseminated formats?
- Does the metadata follow recognised and shared standard schemas?
- Have controlled vocabularies or ontologies been used as far as possible?
- Are links or relationships made available to other resources relevant to understanding the data such as publications or technical reports or software applications?
Re-usable
- Is the data accurate, complete and described in a way that can be easily understood and reproduced?
- Is the dataset given a licence specifying how it may be reused?
- Is it clear from the metadata and accompanying documentation the scientific responsibility and purpose of the data produced?
- Do the data and metadata comply with the quality standards and protocols of the relevant research domain?
In addition to this self-assessment list, a number of online tools for automatically assessing the FAIR compliance of the datasets are also available. None of these are completely exhaustive, but they serve to give you some rough indications about your datasets.
F-UJI is a web service that allows you to evaluate the “FAIRness” of your data automatically if you have a PID (DOI or URL) associated with the dataset you want to evaluate. It is based on metrics developed by the FAIRsFAIR project.
FAIR self assessment tool by the Australian Research Data Commons.
FAIR Enough is also a web service whereby by entering the DOI, URL or handle of an online resource (=your dataset or other research product) you get an evaluation on whether it complies with FAIR principles. It is a service developed by Maastricht University.
FAIR data does NOT mean OPEN data.
The “A” in the acronym “FAIR” indicates that the data must be accessible in some way, but not necessarily open to all.
Remember that data can be confidential but still be managed according to FAIR principles. The guiding principle to follow is “as open as possible, as closed as necessary” depending on the specific needs. Some data may have to remain closed for ethical, privacy or intellectual property protection reasons.
Read more:
What is the difference between “FAIR data” and “Open data” if there is one?
Three camps, one destination: the intersections of research data management, FAIR and Open
Think about how research and innovation could advance faster with the increased reproducibility and transparency enabled by FAIR/Open data and think about the people who could benefit from your data. The first to benefit from FAIR data is you!
“As a scientist, you should treat your data as a love letter to your future self” (Lambert Heller, German National Library of Science and Technology - Nature Index 360o Feb 2019)
Image source: Sara Jones, DCC, University of Glasgow, Open Science Days 2015, 21st & 23rd April, Prague & Brno
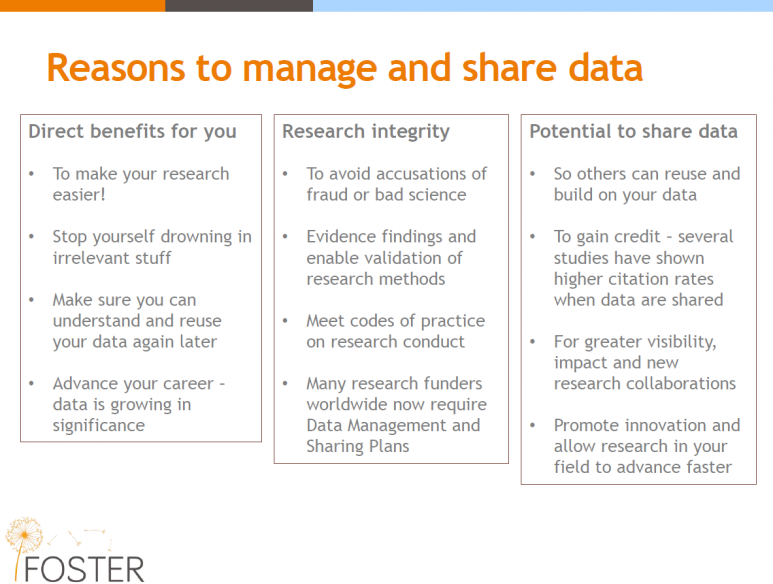
Metadata standards vary from discipline to discipline. Some resources that may be helpful in finding standards for your specific field of research are:
- FAIRSharing, an accurate, informative and informative resource on data and metadata standards, related to databases and data policies.
- Dublin Core, a generic metadata schema
- DCC metadata directory, is a collection of discipline-specific metadata standards
- Data Documentation Initiative o DDI, offers international standards for qualitative and observational data
- RDA alliance has a github repository of subject-specific metadata standards
In some fields of engineering, technology and design, standards for data and metadata are still evolving. It is useful to check with your research community on the development and co-creation of these standards.
Managing research data means consciously and responsibly curating and organising them throughout the research cycle.
Data management strategies must therefore be planned right from the initial stages of research design, then involving all operational phases of data production, collection and analysis, ending with data preservation (long-term archiving) and possibly sharing.
However, each phase involves the consideration of different and very specific aspects of data management.
PLANNING PHASE: during this stage of the project, it will be necessary to identify the type and nature of the data to be generated or reused, and the possible issues involved (privacy, intellectual property, ethical principles).It should be addressed the identification of the metadata to be associated with the data for their correct description and interpretation and it should also be defined the organisation of the data in folders and the rules of file naming. The Data Management Plan should start to be drafted at this stage.
DATA COLLECTION, GENERATION AND PROCESSING PHASE: during the course of this phase of the project, you should define where to store your data and what are the backup strategies. It will also be necessary to keep track of the file "versioning" and to define the modalities of data sharing with possible project partners, providing, if necessary, access systems with authentication and authorisation. In this phase, it will be essential to organise files and folders hierarchically, following a consistent nomenclature and preferring formats for data that are as open and standard as possible.
FINAL PHASE: approaching the end of the project, it will be necessary to assess which data to deposit for long-term preservation, choosing the most suitable and reliable repository in line with FAIR principles. The choice of repository should fall on those that are certified and that guarantee security and control standards. Another aspect to be considered is the association of a licence to the data specifying how it can be reused, where possible.
For more information see the sections "What is a repository and how can I choose one?" and "Criteria for selecting data for long-term preservation".
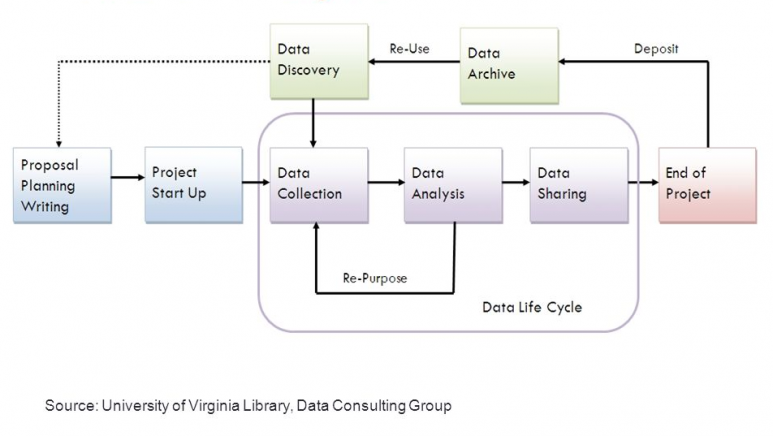
Different research disciplines have different research outputs and it may be necessary to consider various elements when deciding what to select and preserve. Not everything has to be preserved and archived.
Below are some general guidelines that apply to most research disciplines. For your discipline, check the best practices followed by your research community and/or consult the domain expert for Open Science of the Politecnico (mauro.paschetta@polito.it).
Definitely deposit:
- Original data sets, original software code, raw data obtained from the analysis of physical samples, observational data that cannot be regenerated.
- Data sets that are not original and not readily available or available online, which you have permission to share.
- For social science data, include descriptions of studies, code books and summary statistics.
Possibly deposit:
- Intermediate versions of analysis or code if potentially useful to others or if they have been used in publications or theses.
It is not necessary to deposit:
- Incomplete, non-functional or intermediate versions of code that would be of marginal use to others.
- Output files from analyses if the data set and code used to generate the output are deposited and if it is easy enough to regenerate the output from the deposited files.
- Data sets stored and accessible through other institutions or organisations.
- Graphs or tables created from the original data that could be easily regenerated.
Do not deposit:
- Any data containing personal information that identifies human subjects or data that could violate legal contracts.
Exceptions:
- Analysis output files can be deposited if they take a long time to regenerate or are excessively large or cannot be easily recreated from the deposited data set and code.
In the context of research data/outputs, a repository is a digital environment that enables the preservation of research data and other digital outputs in the long term. Essentially, it should offer the following functionalities:
- Stores data securely
- Ensures that data can be found
- Describes data appropriately (metadata)
- Adds licence information
You can deposit data in a generic repository (e.g. Zenodo, Harvard Dataverse, Dryad) or in a subject-specific repository (e.g. IoChem-BD for computational chemistry and materials science, OpenEI for energy, ORNL DAAC for biogeochemistry).
Looking for a repository for your discipline? Try searching on www.re3data.org an oline global registry of research data repositories from all academic disciplines. See a demonstration of a search for data repositories using the re3data directory.
As a good practice and in line with the University Policy on research data management, after depositing in the repository of your choice, we invite you to archive the metadata of your datasets in the PORTO@IRIS institutional repository in the "9. FAIR Data Collection".
OpenAIRE provides a detailed costs guide that will give you an indication of the time, effort and budget required for RDM-related activities, ranging from data archiving, data cleaning, software licence costs to data analysis and archiving in a repository.
Remember that these costs can be budgeted in your financing proposals.
Software is now a fundamental component of research activity. Researchers use software for research activities, they develop their own software as part of the research results and the software itself can be the object of study.
For good scientific practice, research software, just like data, should adhere to FAIR principles to allow full reproducibility and reuse. However, given its evolutionary nature, its composite architecture and its machine-language coding, it requires some special archiving and maintenance practices.
Publishing open source research software is an established practice in science on platforms such as GitHub and GitLab.
Growing community initiatives such as software carpentries help train researchers, who have no specific background in software development or programming, to establish workflows that help them manage, monitor, preserve and, if possible, share or publish research software using task automation tools and version control systems such as Git.
For more information on how to make the software FAIR: